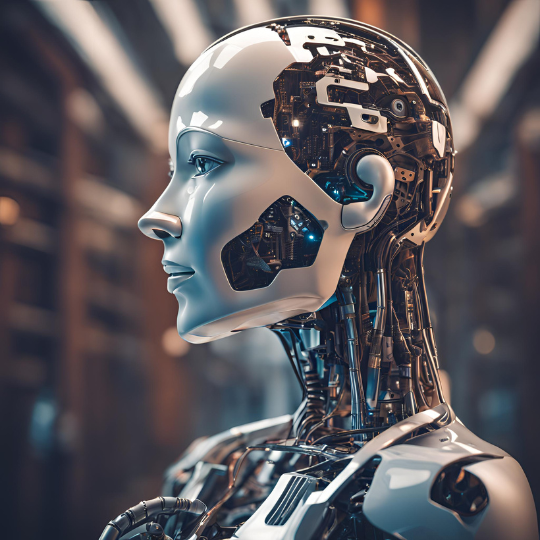
I recently had the chance to join the OxML 2024 program, which brings together people working in machine learning to discuss the latest advancements in AI. During the event, I listened to an inspiring talk by Reza Khorshidi, a machine learning researcher at Oxford and the Chief Product & AI Officer at ELANDI. He spoke about a new concept called “Generation 1st products” in AI, which made me think about how AI is changing the way we work.
As AI continues to evolve, it is clear that traditional approaches, such as Proof of Concept (PoC), are giving way to more meaningful frameworks like Proof of Value (PoV), as companies seek not just technical feasibility but measurable business impact in this rapidly changing landscape.
I’ll briefly summarise the ideas he shared about PoC vs PoV.
Anyone familiar with building products has seen this chart — usually the basis of building a product. Every company wants to build products that are desirable, feasible, and viable.
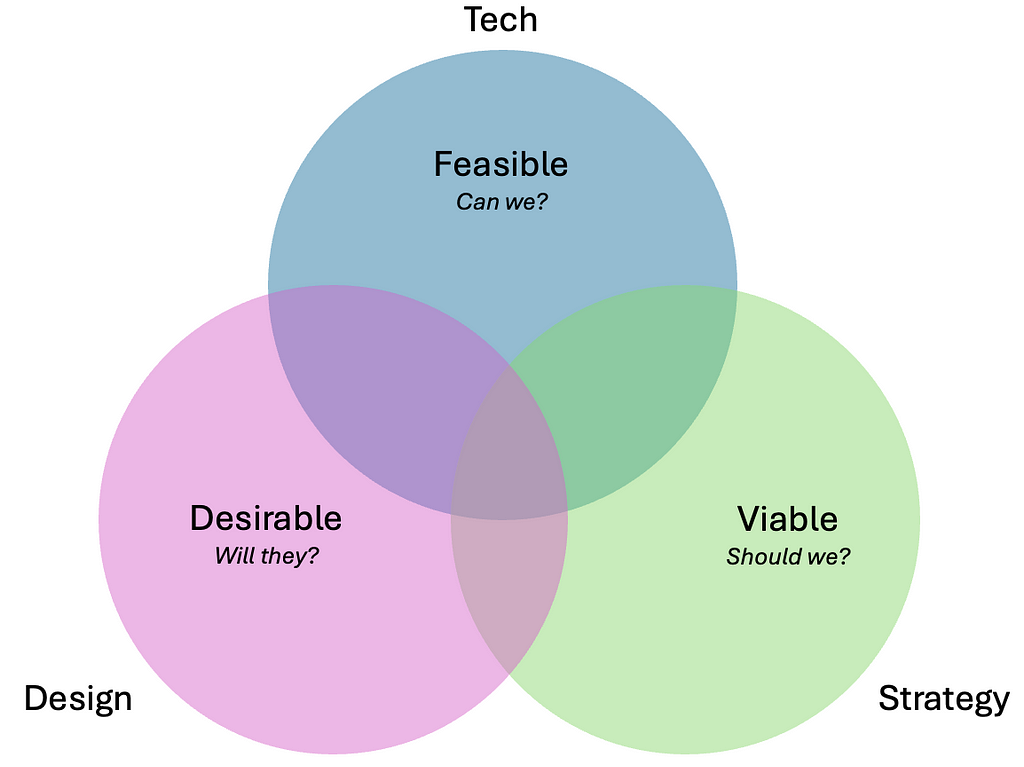
When building a product, we focus on three key questions. Let’s take the example of building an autonomous car:
- Can we use machine learning to develop an autonomous car? (Tech question)
- If we build it, will people buy it? (Design question)
- Can we afford to build it? (Strategy question)
Even if we can technically build the car and there’s demand for it, the real challenge may be whether we can do so without going bankrupt due to the high costs of research and development (Strategy question).
Many of today’s business challenges have become outdated. Problems like text extraction, chatbots, or text summarisation, which once took R&D teams months to solve, can now be quickly addressed using Foundation Models. This represents a major shift in the Generation 1st world, where we can now assume that the technological aspect of these problems is already solved.
Based on this idea, I will examine why PoCs may no longer make sense in the world of AI and why organisations should transition to PoV.
What is a PoC?
A Proof of Concept (PoC) traditionally assesses whether a solution is feasible before full-scale implementation. It’s designed to show that an idea can be implemented effectively and meets the necessary technical requirements. However, as AI solutions grow in complexity — incorporating machine learning, predictive analytics, and natural language processing — PoCs are increasingly seen as insufficient. Companies are now moving toward Proof of Value (PoV), which not only demonstrates technical feasibility but also evaluates the potential business value and impact of AI systems.
What is causing this shift?
PoCs primarily address technical feasibility, focusing on whether a technology or solution can be implemented. However, they often overlook broader AI challenges, such as scalability, long-term value, and real-world impact. While AI models can produce promising results in controlled settings, these outcomes don’t always translate into real business value when scaled.
PoCs are usually time-bound and narrow in scope, aiming for short-term results. However, AI requires continuous learning and adaptation, as many machine learning models improve over time. Their full benefits are realised only after ongoing use and iteration.
Today’s decision-makers are increasingly interested in measurable business value — such as cost savings, revenue growth, or efficiency improvements — beyond just technical feasibility. In many cases, PoCs end before a real return on investment (ROI) can be assessed.
The Rise of Proof of Value (PoV) in AI Projects
The shift from PoC to PoV is essential as organisations seek clear evidence that their AI investments will drive meaningful results. Take document intelligence solutions, for example. A PoV can measure time savings in document processing, and the operational cost reductions these improvements create — metrics that directly impact a company’s bottom line.
According to a recent McKinsey report, while 50% of enterprises claim to have “integrated some form of AI,” only 21% have successfully embedded AI across multiple business units. Many of these projects fail because the AI solution falls short of business expectations.
Similarly, the Intelligent Automation Exchange USA 2023 report surveyed 50 industry leaders on the challenges in proving the value of AI investments and turning PoCs into real-world applications. The biggest challenge identified was visualising the full end-to-end impact of these investments.
Another significant challenge cited in the report was knowing how to quantify AI’s benefits and deciding which metrics to track. Nearly 41% of respondents highlighted cost savings as a crucial metric for proving AI’s value, while 25% emphasised time efficiency gains.
How can companies ensure successful PoVs
When it comes to implementing AI in the real world, we need to make sure it actually provides value to the business.
A study conducted by digital transformation firm Mindtree, which surveyed IT leaders about their AI adoption, revealed that while 85% of organisations have implemented a data strategy and 77% have invested in AI-related technologies, but only 31% have seen a return on their investment.
So, how do companies ensure successful PoVs?
Here are 8 simple strategies I compiled from various talks presented at the OxML 2024 program:
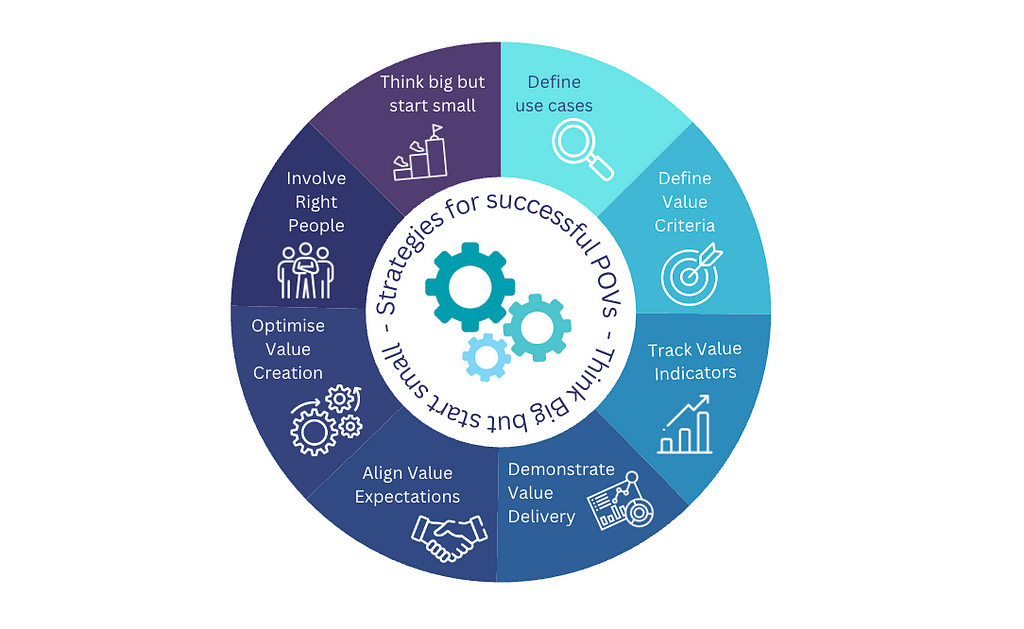
#1 Define use cases
When developing an AI strategy, many companies are focusing on pain points and defining use cases. There must be a shift in mindset and a focus on identifying what success looks like — whether that’s faster document processing, better fraud detection, or more accurate predictions.
Example: A bank identifies the need to speed up loan approvals, focusing on pain points like manual document review and error-prone data entry. They define a specific AI use case for automating document processing to reduce approval times and improve customer satisfaction.
#2 Define value criteria
Define what value means for stakeholders, customers, and users. Value criteria are the specific, measurable, and achievable outcomes that a company wants to achieve with AI solutions. They can be related to revenue, cost, quality, customer satisfaction, or any other relevant metric. The value criteria should align with the business goals and strategy, and prioritise them according to their importance and feasibility.
Example: An e-commerce company defines its value criteria for a recommendation system AI project, setting measurable goals such as increasing average order value by 10% and boosting click-through rates by 15%. This aligns with the business goal of driving revenue growth and customer engagement.
#3 Track value indicators
Value indicators are the key performance indicators (KPIs) that measure the progress and impact of an AI project. They can be quantitative, such as accuracy, speed, or conversion rate, or qualitative, such as user feedback, testimonials, or reviews. These indicators should be monitored regularly, and use them to adjust the project scope, features, and priorities.
Example: A healthcare provider uses AI for predictive patient outcomes and tracks key indicators like prediction accuracy, response time, and patient feedback. Regularly monitoring these KPIs allows the company to adjust the model to improve clinical decision support and patient care quality.
#4 Demonstrate value delivery
Tracking value indicators alone is not sufficient. Companies must also demonstrate how AI projects are delivering meaningful value to stakeholders, customers, and users. Value delivery involves clearly, concisely, and compellingly showcasing the results and benefits of an AI project. This can be achieved through various methods, such as reports, dashboards, demos, or case studies.
Example: A logistics company demonstrates AI project success through dashboards that show reduced delivery times and fewer delays. A case study details how the AI improved route optimisation, illustrating the cost savings and service improvements for stakeholders.
#5 Align value expectation
Value alignment ensures that an AI project is consistent with the business environment and objectives, aligning with the expectations of stakeholders, customers, and users. AI projects do not operate in isolation; they are part of a broader business context and both influence and are influenced by factors like market trends, customer behaviour, and competitor actions.
Example: A retail company implements an AI-driven demand forecasting tool but aligns the project goals with the company’s seasonal marketing strategies and customer buying trends. Regular updates with stakeholders ensure that the project remains aligned with both market demands and customer expectations.
#6 Optimise value creation
Value optimisation involves enhancing, scaling, or extending AI projects based on data and feedback. AI projects are never truly complete — they continuously evolve, learn, and improve. To maximise their value, these projects require ongoing optimisation, which may include boosting performance, reliability, usability, and accessibility, or reducing costs, risks, and limitations.
Example: A SaaS provider continuously gathers user feedback on an AI-powered support chatbot, optimising it for better accuracy and faster response times. This ongoing refinement helps reduce support costs while improving user satisfaction and retention.
#7 Involve the right people
Don’t leave AI projects just to the tech team. Cross-team collaboration ensures that the PoV covers all aspects of the business, not just technical performance. If necessary, up skill the team to boost value.
Among the respondents from Mindtree survey , 44% reported hiring top talent from external sources, 30% have formed partnerships with academic institutions, and 22% organise hackathons to tackle new challenges.
Example: An insurance company includes its risk, compliance, and IT teams when developing an AI model for fraud detection. By involving these departments, they ensure the AI solution adheres to regulatory standards, minimises risk, and effectively addresses both technical and business needs.
#8 Think Big but Start Small
A company may have a clear vision of how AI can transform its business processes, but it’s wise to start with a small, manageable use case. Starting small enables quick wins while ensuring the solution can scale to meet future demands.
Example: A global manufacturer envisions an AI solution to monitor equipment health across all plants worldwide. However, they first implement it in one facility to demonstrate quick wins in reducing equipment downtime. After success in the pilot, they scale it company-wide, informed by insights gained from the smaller rollout.
The future of AI projects
In the AI-driven world, where technical feasibility is often not the most pressing concern, PoC is no longer sufficient to justify large-scale investments. Proof of Value offers a more robust framework, focusing on real-world outcomes, measurable business value, and strategic alignment. The transition from PoC to PoV allows companies to de-risk AI projects, ensure stakeholder buy-in, and achieve scalable success.
By focusing on value rather than just feasibility, organisations can better position themselves to harness the true power of AI, ensuring that their investments lead to meaningful business transformations.
Resources
https://www.linkedin.com/advice/3/how-do-you-prove-business-value-ai-delivered
https://www.ltimindtree.info/gen-ai
https://www.intelligentautomation.network/
Why PoC Is Becoming Obsolete in the AI Era? was originally published in Towards Data Science on Medium, where people are continuing the conversation by highlighting and responding to this story.
Originally appeared here:
Why PoC Is Becoming Obsolete in the AI Era?
Go Here to Read this Fast! Why PoC Is Becoming Obsolete in the AI Era?