Key recommendations for how to succeed with data science consulting projects and build lasting client relationships

Introduction
I am not ashamed to say it: Data science consulting is not always easy! It can be brutal — especially at senior levels when you need to generate sales to stay in the game. Even if keeping your clients happy is at the top of your priority list, it isn’t always a trivial exercise to do that with data science projects.
Reflecting on over a decade of delivering data science and data engineering projects — the majority of which has been as a consultant — I have seen projects deliver incredible value to clients, but I have also seen projects stumble and falter, delivering mediocre results, often due to poor planning, misaligned expectations and technical difficulties.
It is clear that successful data science consulting isn’t just about being a Python and R wizard — acing Hackerrank data science programming competitions; it’s much deeper than that, being able to blend strategy, technology and analytics in project deliverables. In this article, I will draw insights from both successful and challenging projects and illustrate how data science consulting intersects with management, IT and analytics consulting. By understanding the differences and similarities between these more traditional and established consulting roles it becomes easier to see how we can deliver lasting and impactful solutions in our data science projects.
Similarities and Differences with Traditional Consulting Roles
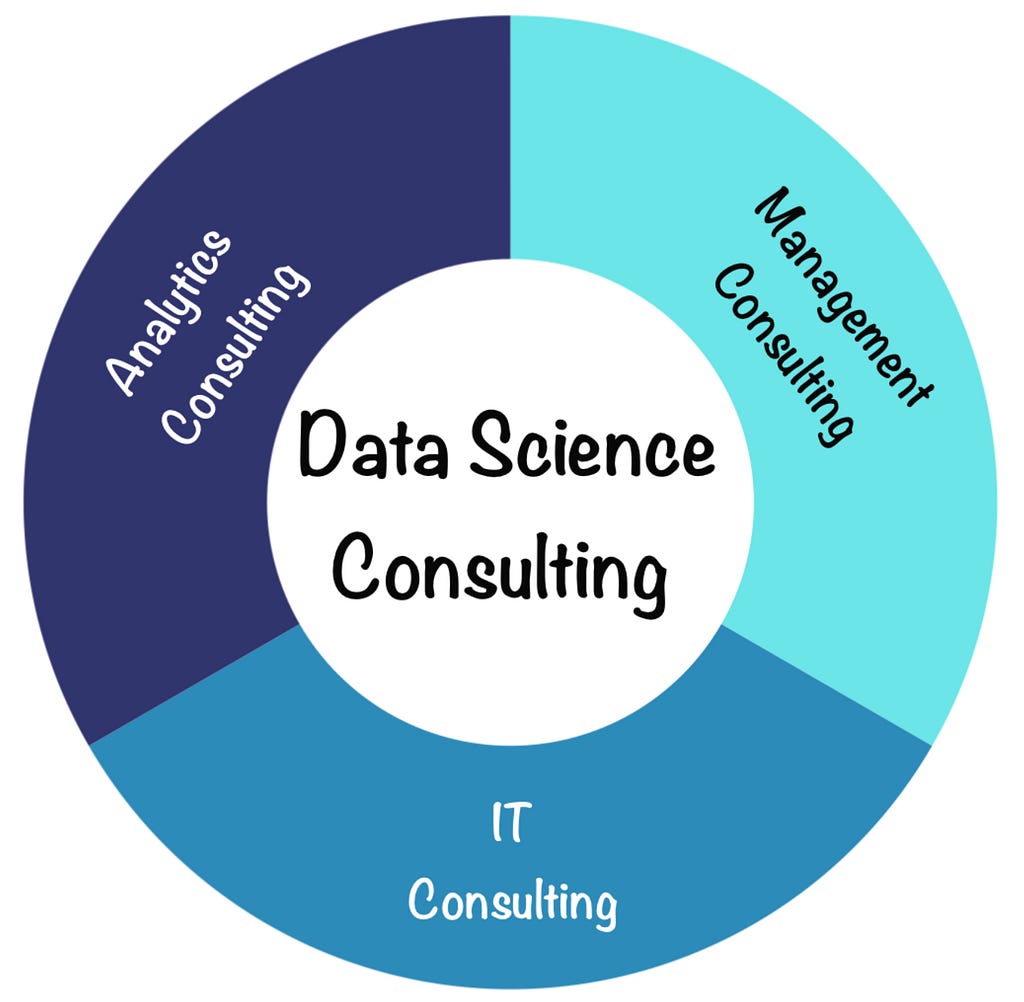
Management Consulting vs. Data Science Consulting
Management consulting projects and data science consulting projects usually share many of the same characteristics. They both try to enhance business performance and often deliver projects of strategic importance. They also usually involve senior executives — at least as project owners — and they both require detailed upfront planning. However, while I find that management consulting projects usually follow a traditional waterfall approach, data science consulting can benefit more from a hybrid approach that combines some of the structured planning from waterfall with agile iterations.
Regarding deliverables, management consulting projects will typically produce strategic plans, organizational assessments or pricing recommendations, and they are often contained in a power point deck or and an excel workbook. This is in contrast to data science projects, where the main deliverables will typically be predictive models, data pipelines and dashboards.
The feedback cycles are also different, while the traditional management consulting projects will typically have planned periodic feedback, the data science consulting projects are more successful with continuous stakeholder involvement and iterative feedback cycles.
IT Consulting vs. Data Science Consulting
IT consulting and data science consulting both require strong technical expertise and an understanding of IT infrastructure, and some people might argue that data science consulting is a subcategory of IT consulting. I don’t subscribe to that belief, but the two fields have a considerable overlap.
While IT consulting projects produces system architecture designs, implementation plans and more core system software development, data science consulting is more centered around generating predictive models, the pipelines that feed the models and data-driven insights.
Additionally, data science projects are often very closely connected to the business side of the organizations and will in many cases have leaders outside of IT as project owners and sponsors. For example, you can find yourself delivering directly to the CMO (Chief Marketing Officer) or the CDO (Chief Data Officer) instead of the CIO (Chief Information Officer) or CTO (Chief Technology Officer), as is often the case with more IT-driven projects. This means that your approach and method needs to be tailored differently than with pure IT projects, and you often need to make more considerations regarding continuity, operations and maturity of the organization. (The CIOs and CTOs will already typically have more elaborate systems in place to deal with the above issues.)
However, as data science has matured over the last years, we have seen fields like MLOps evolve significantly, and also a trend in projects to develop and write code closer to how modern software development is undertaken. I see this as a natural part of the evolution of data science towards a more professional and structured field.
Analytics Consulting vs. Data Science Consulting
Lastly, comparing analytics to data science consulting we find that they both share a focus on data and typically involve shorter project durations with targeted deliverables. They also emphasize rapid iterations and continuous refinement based on data and feedback. However, analytics consulting often addresses specific business questions with more narrow scopes, while data science consulting integrates a broader strategic alignment and more complex models.
The classical deliverables within analytics consulting would usually be data analysis reports, data marts and dashboards, whereas data science consulting deliverables — as we have mentioned previously — are more centered around pipelines, models and strategic and integrated data products.
Finally, how the projects are evaluated if usually also different. While the analytics projects are assessed more around the analysis accuracy and insights, the data science projects will typically in addition be measured on predictive accuracy and return on investment.
Notes to the critical reader: The above descriptions are by no means set in stone and are meant to show the general differences and similarities between the various classes of consulting. In many cases they will obviously diverge or merge more than above.
Key Insights for Successful Data Science Consulting
Given the above similarities and differences between data science consulting and other classes of consulting, it is natural to ask how we might adapt our approach to ensure the long term success and viability of our projects. Apart from the obvious elements such as quality deliverables, timely project delivery and strong stakeholder management, what are the other components that need to be in place to succeed?
Ensuring Robust Data Products
While management consulting typically focuses on immediate organizational changes and one-off deliverables, data science consulting requires a long-term perspective on robustness and sustainability. This has a couple of consequences, and you can and will be judged on the continued performance of your work and should take steps to ensure you deliver good results not just at the moment of handover, but also potentially for years to come. (This is similar to IT consulting, where ongoing performance and maintenance are essential.)
For instance, I’ve built data products that have been in production for over 6 years! I have seen the direct effects of having data pipelines that are not robust enough, leading to system crashes and erroneous model results. I have also seen model variables and labels drift significantly over time, leading to degradation of system performance and in some cases completely wrong insights.

I know that this is obviously not the most sexy topic, and in a project with tight budgets and short timelines it can be hard to make the argument to spend extra time and resources on robust data pipelines and monitoring of variable drift. However, I strongly compel you to spend time with your client on these topics, integrating them directly into your project timeline.
– Focus on long-term sustainability.
– Implement robust data pipelines.
– Monitor for model and variable drift continuously.
I have written about one aspect of data pipelines (one-hot encoding of variables) in a previous article that aims to illustrate the topic and provide solutions in Python and R.
Documentation and Knowledge Transfer
Proper documentation and knowledge transfer are critical in data science consulting. Unlike analytics consulting, which might involve less complex models, data science projects require thorough documentation to ensure continuity. Clients often face personnel changes, and well-documented processes help mitigate the loss of information. I have on multiple occasions been contacted by previous clients and asked to explain various aspects of the models and systems we built. This is not always easy — especially when you haven’t seen the codebase for years— and it can be very handy to have properly documented Jupyter Notebooks or Markdown documents, describing the decision process and analysis. This ensures that any decisions or initial results can easily be traced back and resolved.
– Ensure thorough documentation.
– Use Jupyter Notebooks, Markdown documents or similar.
– Facilitate knowledge transfer to mitigate personnel changes.
Building End-to-End Solutions
Building end-to-end solutions is another key consideration in data science consulting. Unlike analytics consulting, which might focus on delivering insights and reports, data science consulting needs to ensure the deployability and operationalization of models. This is similar to IT consulting, where integration into existing CI/CD pipelines is crucial.
I’ve seen companies waste years from the development of a model to its production deployment due to personnel changes and unfinished integration tasks. If we had insisted on seeing the project through to full production ready status, the client would have had the full benefits of the model much earlier than they ended up doing. This can be significant when project costs can be in the millions of euros.
– Build deployable models.
– Ensure operationalization.
– Integrate into existing CI/CD pipelines.
Visual Artifacts
Including visual artifacts, such as dashboards or widgets, helps demonstrate the value created by the project. While management consulting deliverables include strategic plans and assessments — usually in the form of a one-y power point deck — data science consulting benefits from visual tools that provide ongoing insights into the impact and benefits the solution has. These artifacts serve as reminders of the project’s value and help in measuring success, similar to the role of visualizations in analytics consulting.
One of my most successful projects was when we built a pricing solution for a client and they started using the dashboard component directly in their monthly pricing committee meetings. Even though the dashboard was only a small fraction of the project it was the only thing that management and the executives in the company could interact with and thus provided a powerful reminder of our work.
– Create visual artifacts like dashboards.
– Demonstrate project value visually.
– Use artifacts to measure success and stay relevant to the client.
Evaluating Organizational Maturity
Evaluating organizational maturity before building the project is essential to avoid over-engineering the solution. Tailoring the complexity of the solutions to the client’s maturity level ensures better adoption and usability. Always remember that when you are finished with the project, ownership usually shifts to internal data scientists and data engineers. If the client has a team of 20 data scientists and a modern data infrastructure ready to integrate your models directly into their existing DevOps, that’s amazing, but frequently not the case. Consider instead the scenario where you are developing a tool for the company with 20 employees, a fresh a data scientist and and over worked data engineer. How would you adapt your strategy?
– Assess organizational and analytical maturity.
– Avoid over-engineering solutions.
– Tailor complexity to client readiness.
Following Best Practices in IT Development
Following best practices in IT development is becoming increasingly important and often required in data science consulting. Unlike analytics consulting, which might not involve extensive coding, data science consulting should stay true to software development practices to ensure scalability and maintainability. This is similar to modern IT consulting, where writing modular, well-documented code and including sample data for testing are essential practices.
This also ties back to the previous point around documentation and knowledge transfer. Properly documented and structured code, packaged into easy to install software packages and libraries is much easier to maintain and manage than 1000s of lines of spaghetti code. When personnel changes occur, you will be in a much better spot if the code has been properly developed.
– Follow IT development best practices.
– Write modular and well-documented code.
– Include sample data for testing.
I want to end this article with video of Steve Jobs talking about consulting. He clearly doesn’t have too much sympathy for traditional consultants, however I feel that as data scientist consultants we need to be more true to his ideas around really taking ownership of the advice and products that we build. We are measured not just on the successful completion of the project but the ongoing and lasting value we create.
Conclusion
Data science consulting is an exciting and complex profession that draws from management, IT, and analytics consulting. By understanding the similarities and differences and applying best practices from each, you can deliver successful data science consulting projects that drive long term value for your clients. I believe this is a necessity if you want to build a successful data science consulting business. My personal experiences highlight the importance of robust solutions, thorough documentation, end-to-end deliverables, visual artifacts, evaluating organizational maturity, and following IT development best practices in ensuring the success of data science consulting projects.
Thanks for reading!
How to Deliver Successful Data Science Consulting Projects was originally published in Towards Data Science on Medium, where people are continuing the conversation by highlighting and responding to this story.
Originally appeared here:
How to Deliver Successful Data Science Consulting Projects
Go Here to Read this Fast! How to Deliver Successful Data Science Consulting Projects