Fully utilising the power of Google LLM and your private knowledge
· The Problem
· The Solution
· Use Case
· Step by Step Guide
∘ Step 1: Environment Setup
∘ Step 2: Prepare Private Knowledge and store them into Google Cloud Storage (low code)
∘ Step 3: Create Chatbot and the Data Store sitting behind the Chatbot (no code)
∘ Step 4: Test the Chatbot (no code)
∘ Step 5: Publish / Integrate your Chatbot (low code)
∘ Step 6 (Optional): Publish it through a Beautiful Application (low code)
· What makes this “magic”?
· Some Observations
· Wrap-up
· Enjoyed This Story?
The Problem
You might have been familiar with AI chats powered by Large Language Model (LLM) such as OpenAI ChatGPT or Google Bard. And you might have noticed one thing — these LLMs have extensive general knowledge about the world, but might not give you satisfactory answers when you ask about a very specific or professional area, especially if the knowledge of this area is not that publicly available or sharable.
Have you thought about “giving” your private knowledge to LLM and creating your own Chatbot?
Do you know this can be done within 5 minutes with no code or low code?
The end product will be like this:
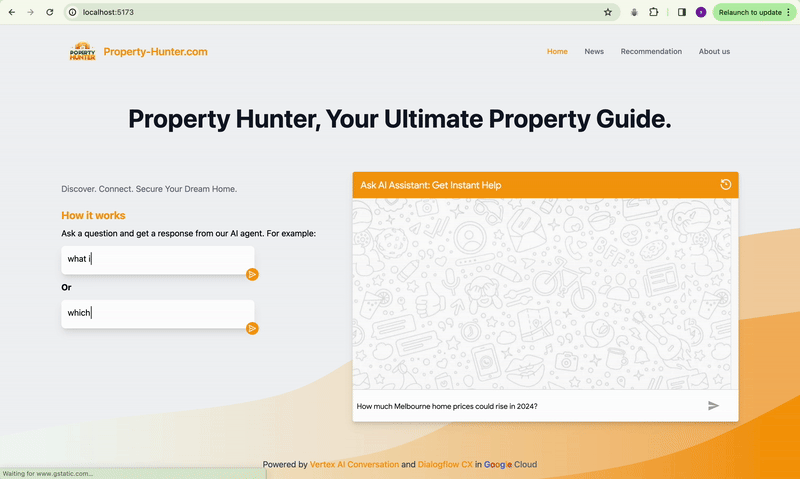
The Solution
During the Asia Pacific Google Cloud Applied AI Summit, Alan Blount from Google shared an interesting idea of achieving this using Google Cloud Vertex AI Search and Conversation, which I found pretty attractive to try out.
The idea is simple, first put a corpus of private knowledge documents onto Google Cloud Storage:
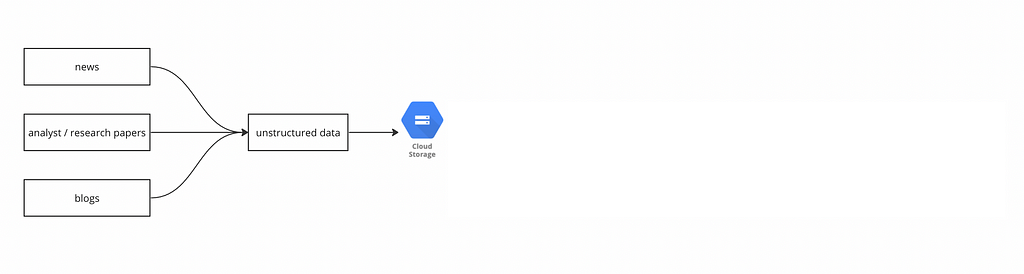
then create a Data Store, and import the documents from the Cloud Storage into the Data Store:
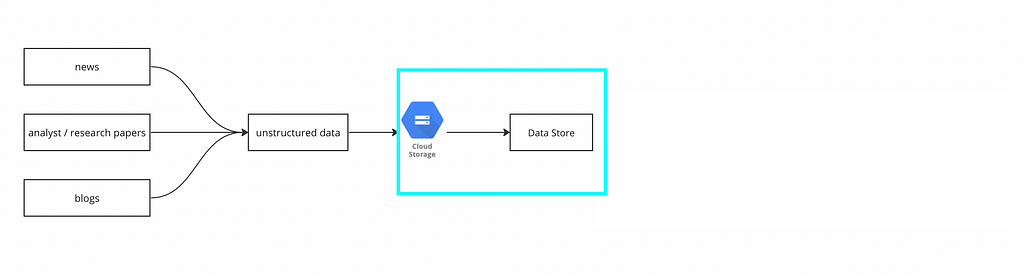
finally plug that Data Store into Dialogflow CX:

then we are done!
We can test Chatbot like this:

And if we want to publish it through a beautiful application, Google provides a public git repo for a Chat App that we can utilise. With a bit of coding knowledge, we can plug the link of the Dialogflow Chatbot into the Chat App, and customize the interface like this:

OR this:
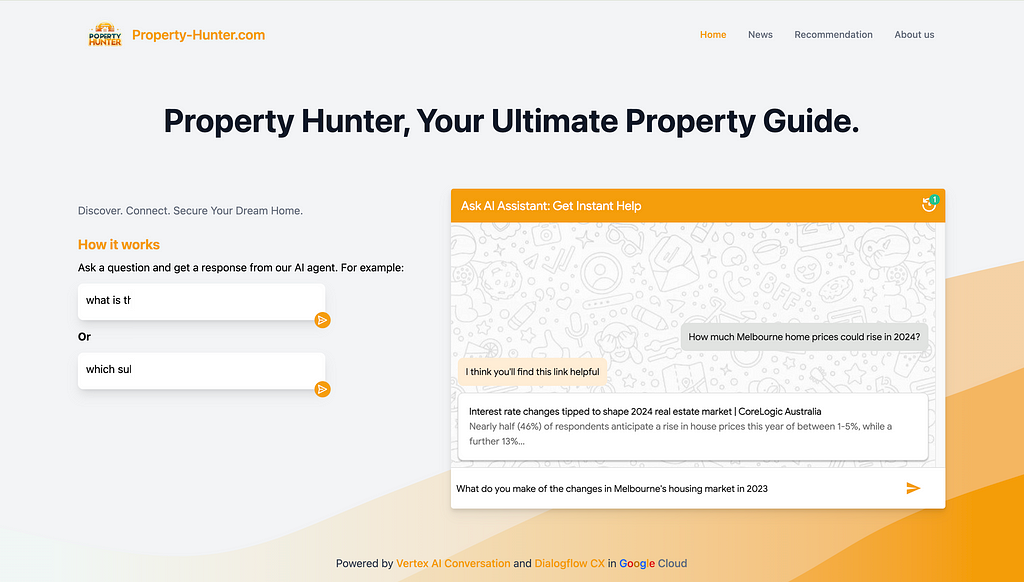
Use Case
In this case, assuming I am the owner of an ecommerce website. I would like to create a Chatbot, so my users can ask specific questions regarding anything about this website (price, product, service, shipping, etc.) as they are in the store. The Chatbot will be supplied with the “private knowledge” and ground its answers to the contents of the website.
Given I am not actually owning an ecommerce website, I will take a workaround to crawl contents from an existing website available on the Internet. This is tricky because most websites are anti-scraping as specified in their terms of use, and it could be illegal to scrape ecommerce websites such as Amazon, eBay, Alibaba, etc.
ChatGPT provided me with a perfect option —
Books to Scrape (https://books.toscrape.com/). A simulated bookstore specifically designed for web scraping practice. It offers a straightforward structure for scraping book details like title, price, and rating.
In this use case, I would assume I am the owner of this Books to Scrape website, and create the Chatbot based on it.
Step by Step Guide
This might look a bit lengthy at first glance because it covers every detailed step that you will need. Once you have run through, you can get the same done within 5 minutes.
Step 1: Environment Setup
The tool we are going to use is sitting on Google Vertex AI and we will need a Google Cloud Platform (GCP) account.
Google has a free-tier program to provide new Google Cloud Platform (GCP) users with a 90-day trial period that includes $300 as free Cloud Billing credits.
Follow the tutorial here to set up the free Google Cloud account.
After you have set up Google Cloud account and can access the console, create a storage bucket (step-by-step guide here) for the next step use.
Step 2: Prepare Private Knowledge and store them into Google Cloud Storage (low code)
As mentioned above, the private knowledge in this case will be the contents sitting on the book store website.
For owners of ecommerce websites, all you need to do is to provide the website URLs, and Google can automatically crawl website content from a list of domains you define.
Given I am not a real owner, I will resolve this by scrawling. Alan Blount from Google provided a very useful notebook to achieve this. All the code snippet does is to scrawl webpages from the website that you specified and store them in a Google Cloud Storage bucket that you specified.
This is all you need to do:
2.1 Save a copy of the notebook in your own drive
Recall that in step 2 you have created a new Google account when you registered for Google Cloud? Your Google account will have Google Drive and you can save a copy of this notebook to your drive.
Select “Save a copy in Drive” option from the dropdown menu of “File”
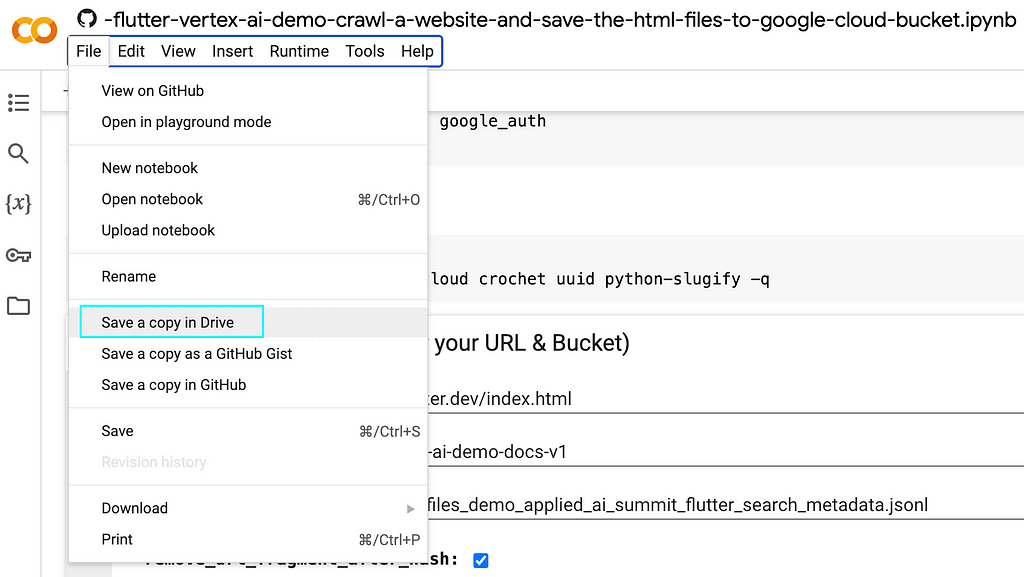
Then if you go to Google Drive, you will be able to see the notebook you created. Feel free to rename it according to your need.
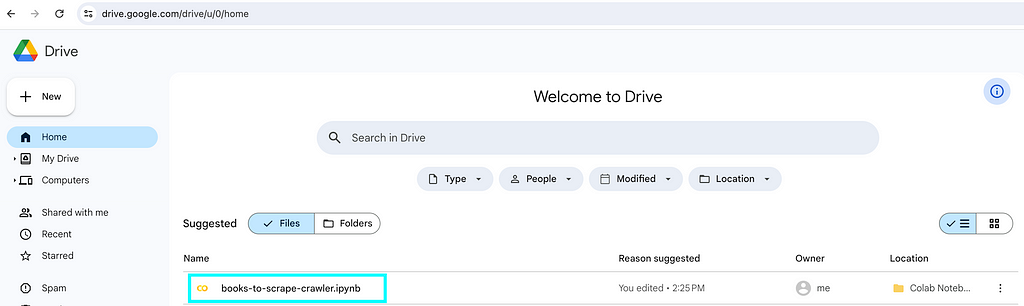
2.2 On your own notebook, locate the below and specify
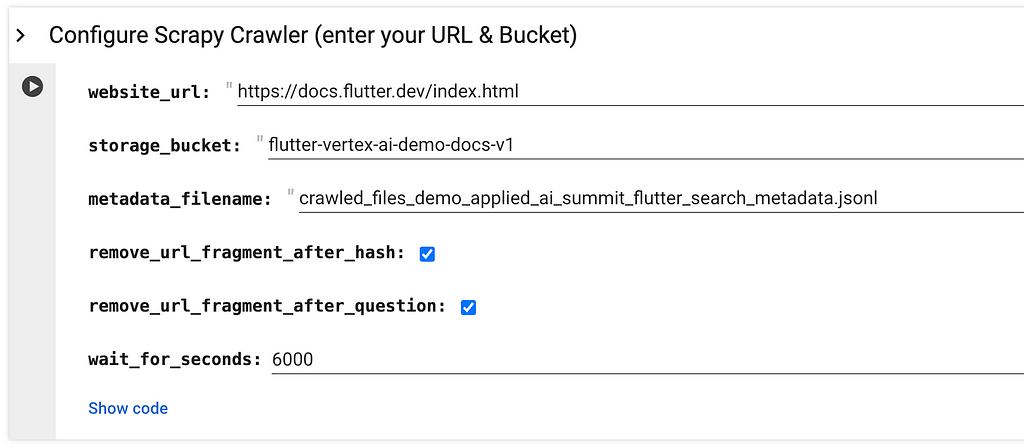
website_url refers to the website page URL that you would like to scrawl.
storage_bucket refers to the Google Cloud Storage that you created in above step 1.
metadata_filename refers to a json file that will be created and stored together with the webpages. You might want to make it relevant to your website by changing applied_ai_summit_flutter_search to something that can describe your use case.
This is my version:
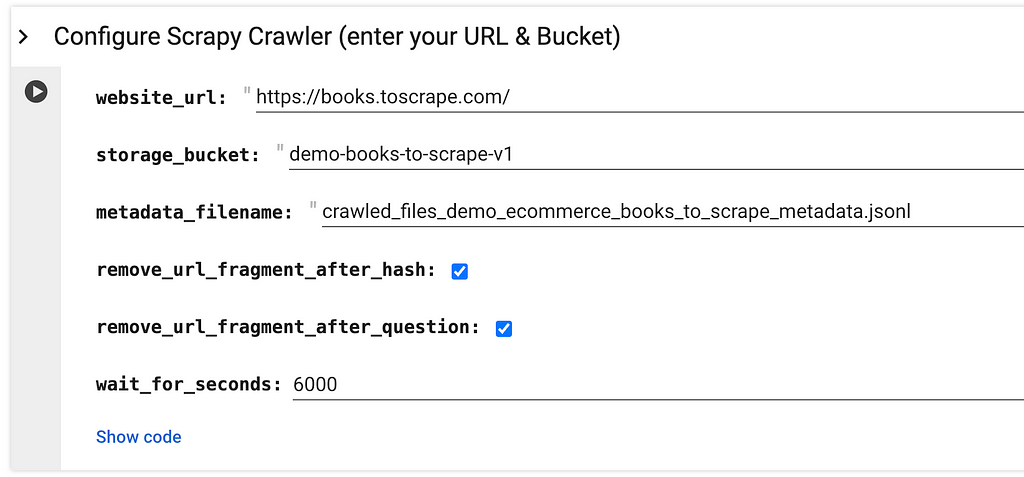
2.3 Run all
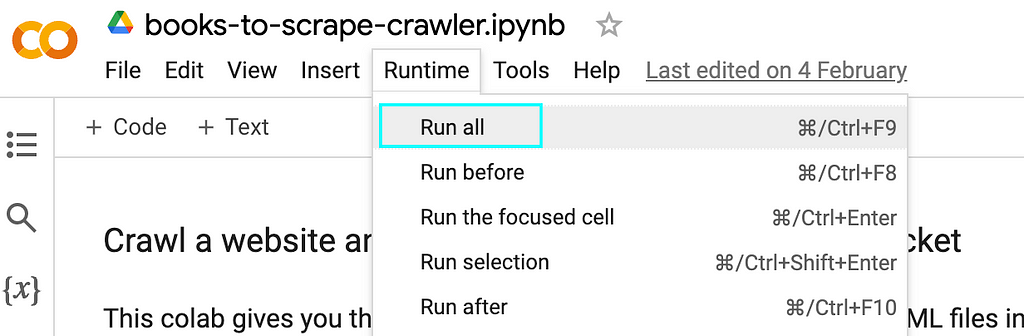
2.4 When it prompts you to authenticate the Google Colab notebook to access your Google credentials, click “Allow” -> “Continue”

Then the script should run through and show the progress of the scrawling at the bottom, just like this:
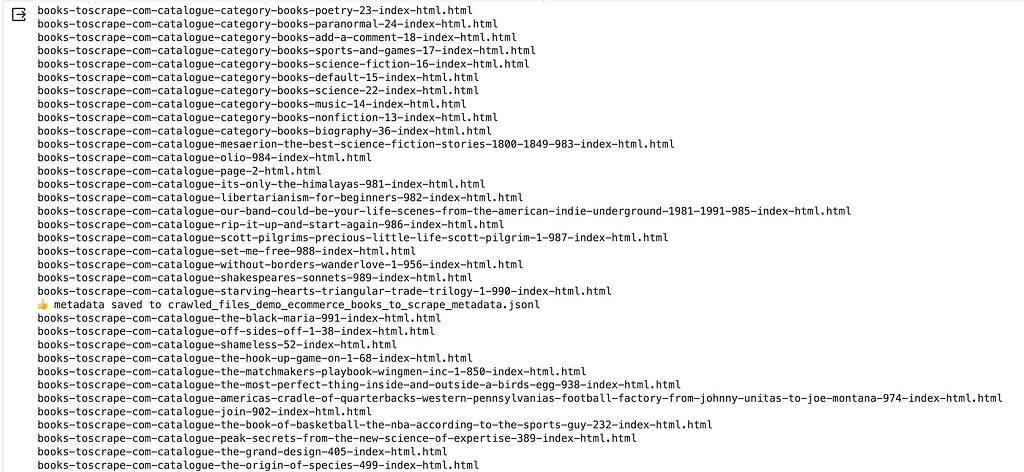
And if you refer to your Google Cloud storage bucket, you will see these html files get scrawled and stored properly within your bucket:
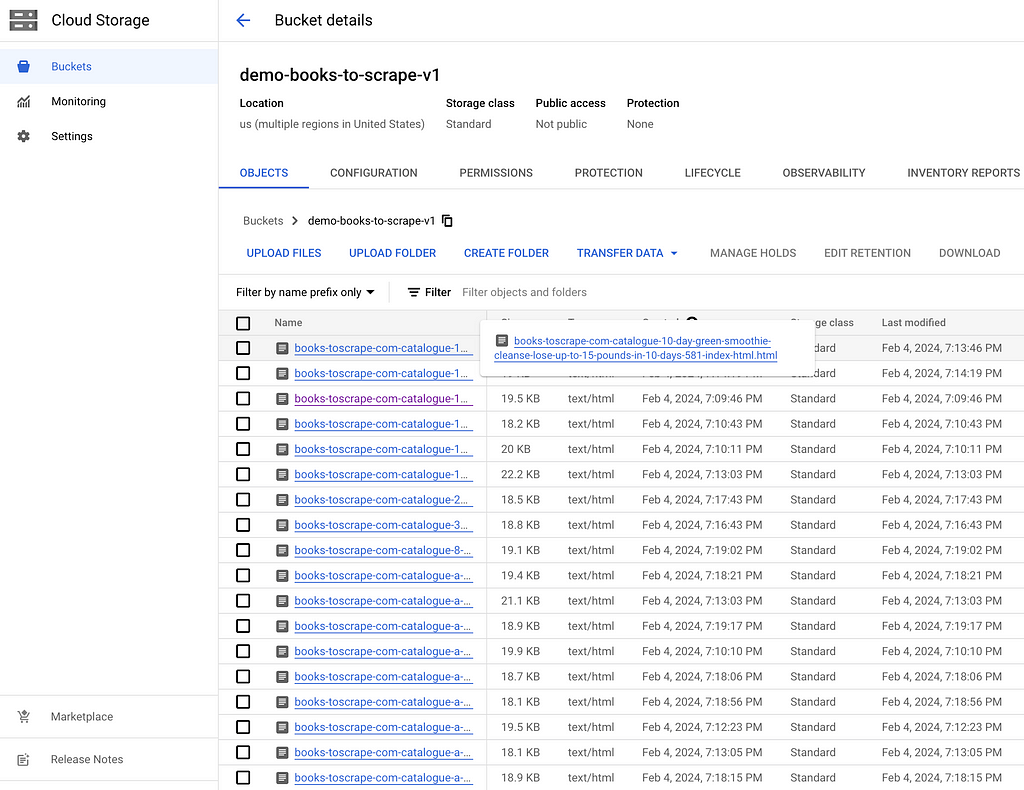
One thing to notice is that the code snippet is not designed for every use case, and you might need some slight tuning of the codes to achieve your goal.
For example, in my case, I tuned the code a bit by changing
blob.upload_from_string(html_string)
into
blob.upload_from_string(html_string, content_type='text/html')
By default the html_string will be uploaded as text/plain . By changing into text/html , I would like to enable this HTML contents to show properly in a later stage.
You can tune the code as much as you like.
Step 3: Create Chatbot and the Data Store sitting behind the Chatbot (no code)
Go to Google Cloud Console (https://console.cloud.google.com/) and type “search and conversation” as the service:

Create “NEW APP”:

Select “Chat”:
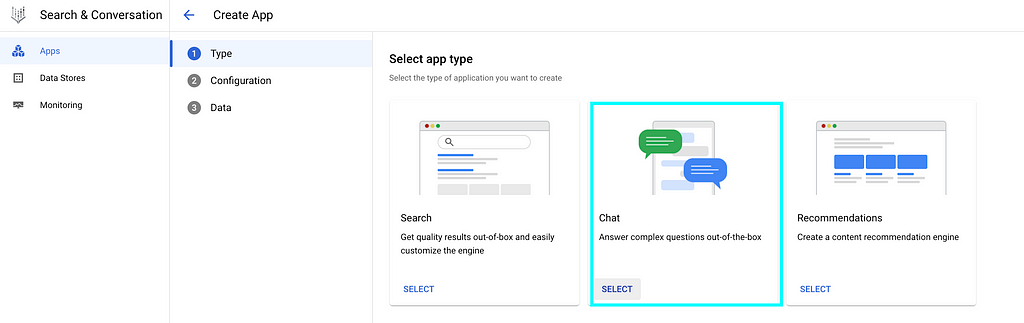
Provide your “Company name” and “Agent name”. Note that the “Agent name” here will be the name of the Chatbot, you might want to put a good name for your users.
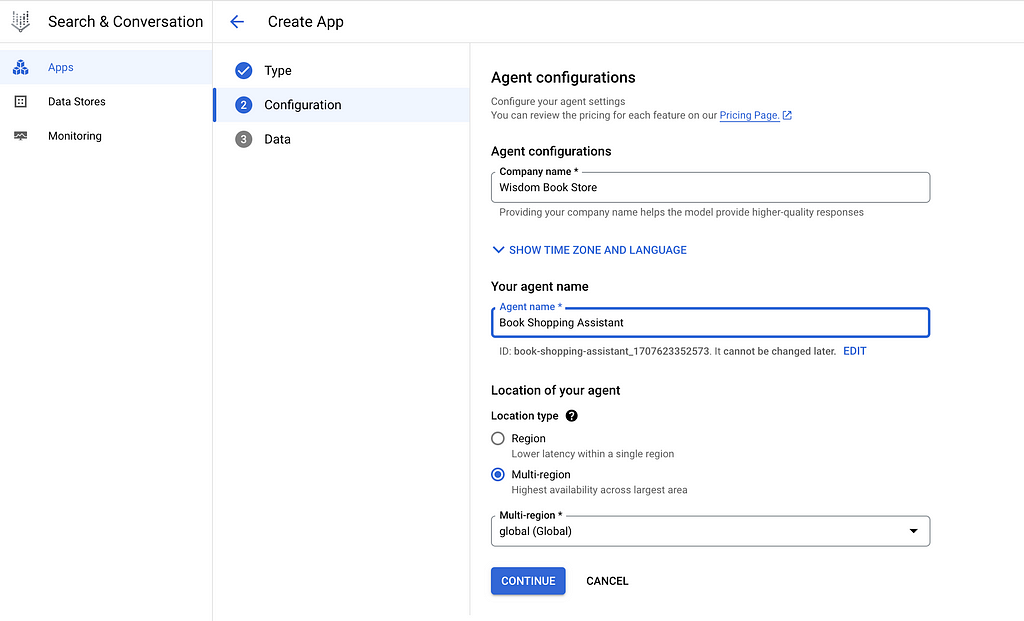
At this “Data” page, select “CREATE NEW DATA STORE”:
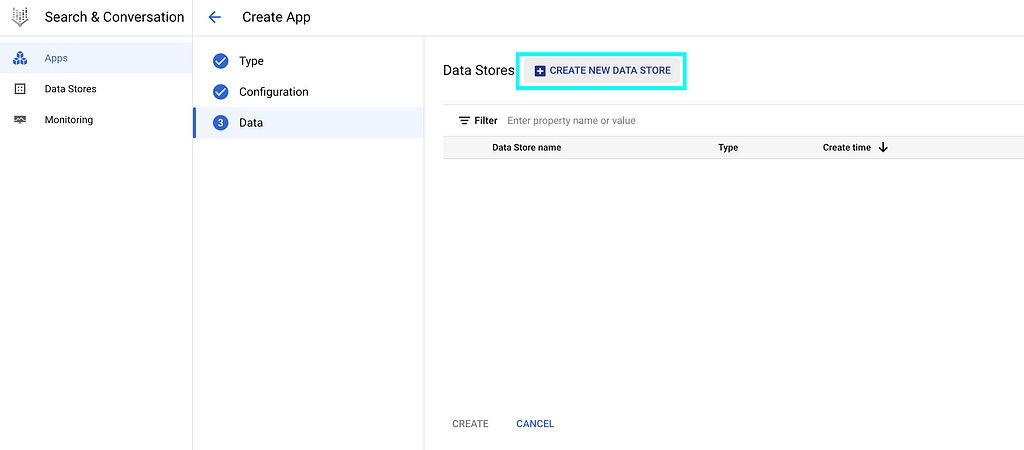
For owners of ecommerce websites, select “Website URLs” and provision your website URLs
As I have scrawled the website contents into Cloud Storage, we can select “Cloud Storage” here:
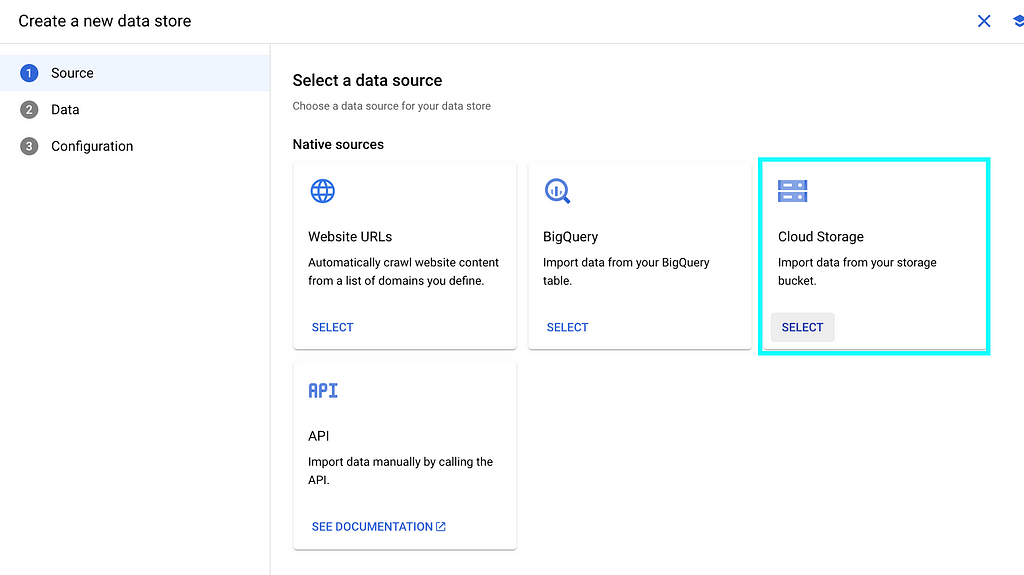
Specify the Cloud Storage bucket name, and select “Unstructured documents” in below:
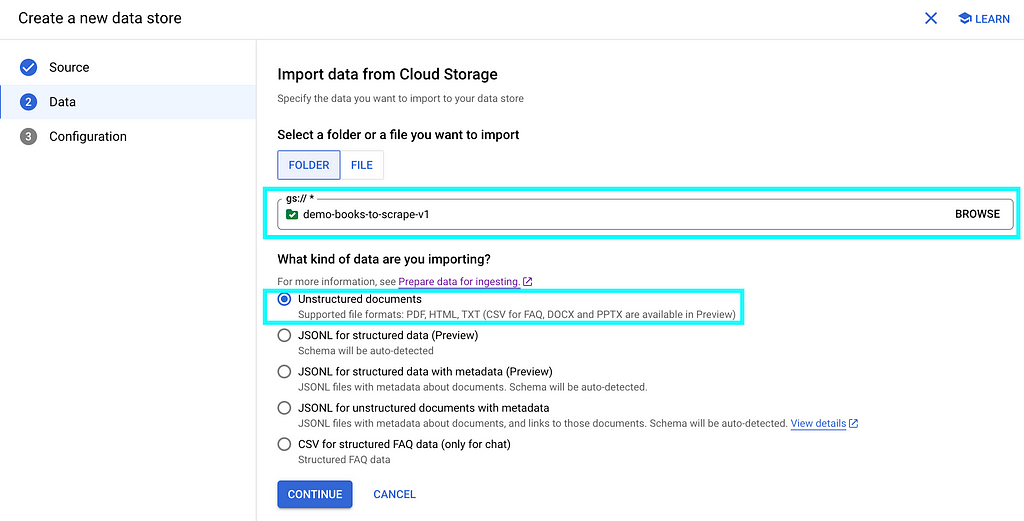
Give your data store a name, then “CREATE”
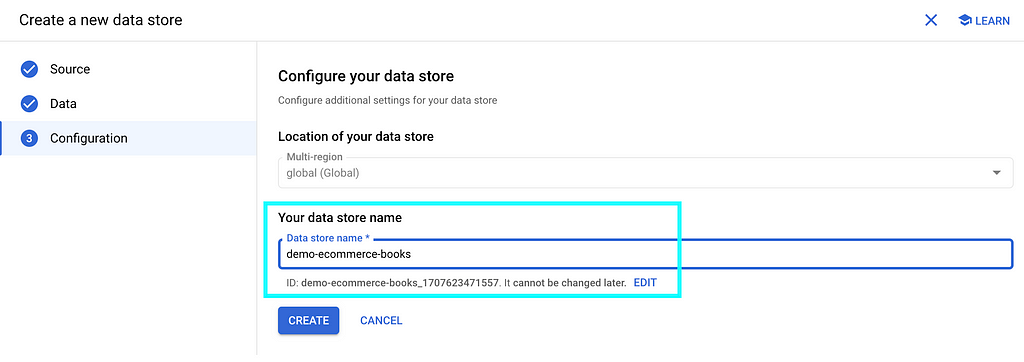
You will see your data store listed, then “CREATE”

Your data store will be created as below
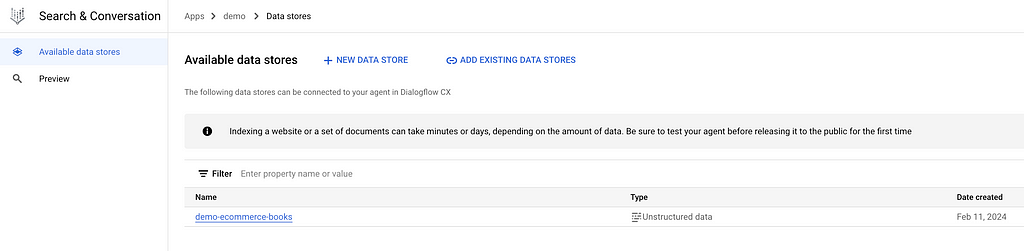
If you click into it, you will see your data store is “processing data” by importing documents from the Cloud Storage bucket that we specified earlier:

If we click the “ACTIVITY” tab, we can see the import is in progress:

Import will take minutes to hours depending on the number of documents in your Cloud Storage bucket.
In my case, I have over 1,000 files and it finishes within minutes.
After import is completed, the status as highlighted has changed:

And if you switch back to the “DOCUMENTS” tab, you will see the list of files imported into the data store:

That means you’ve got all the materials and you are ready to cook!
Step 4: Test the Chatbot (no code)
In step 3 above, we have already created a Chatbot app as well as the data store sitting behind it.
Click “Apps” on the top:

You will see the Chatbot you created in the previous step 3:

If you click into the Chatbot name, you will be directed to the Dialogflow CX page like below:
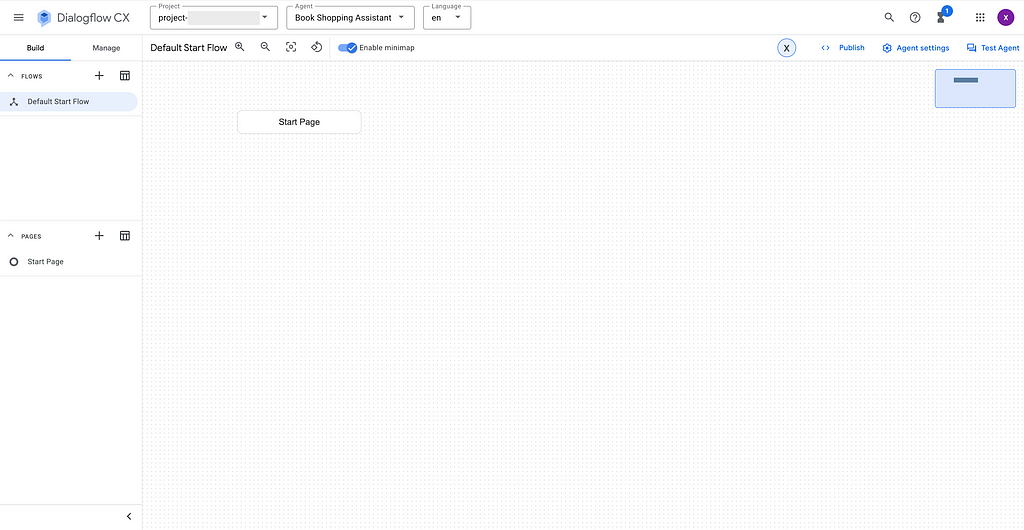
To test the Chatbot, select “Test Agent” in the right up corner:
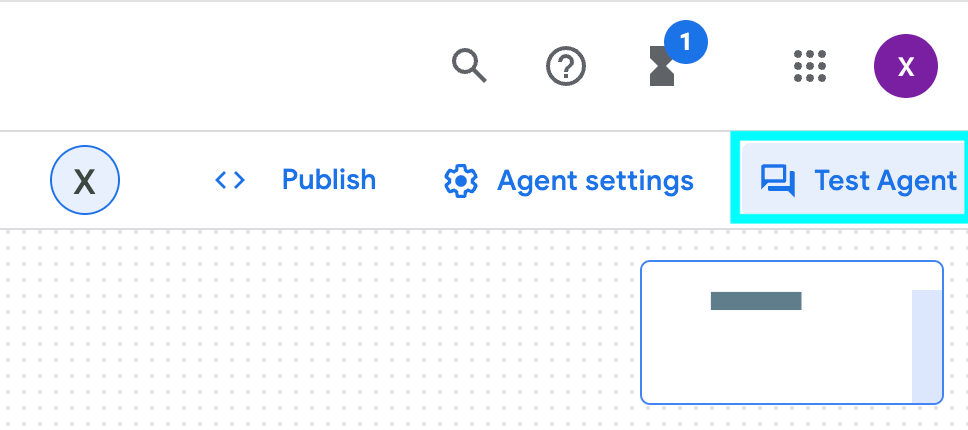
And the dialogue box will pop up:
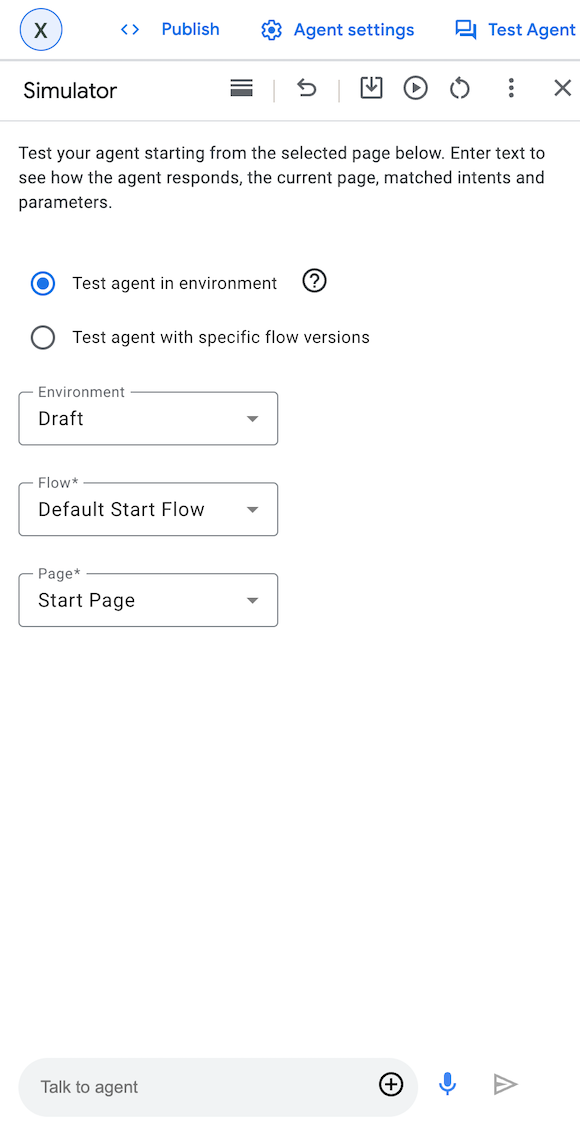
You can start the conversation by saying “hi” and start asking questions to the Chatbot:
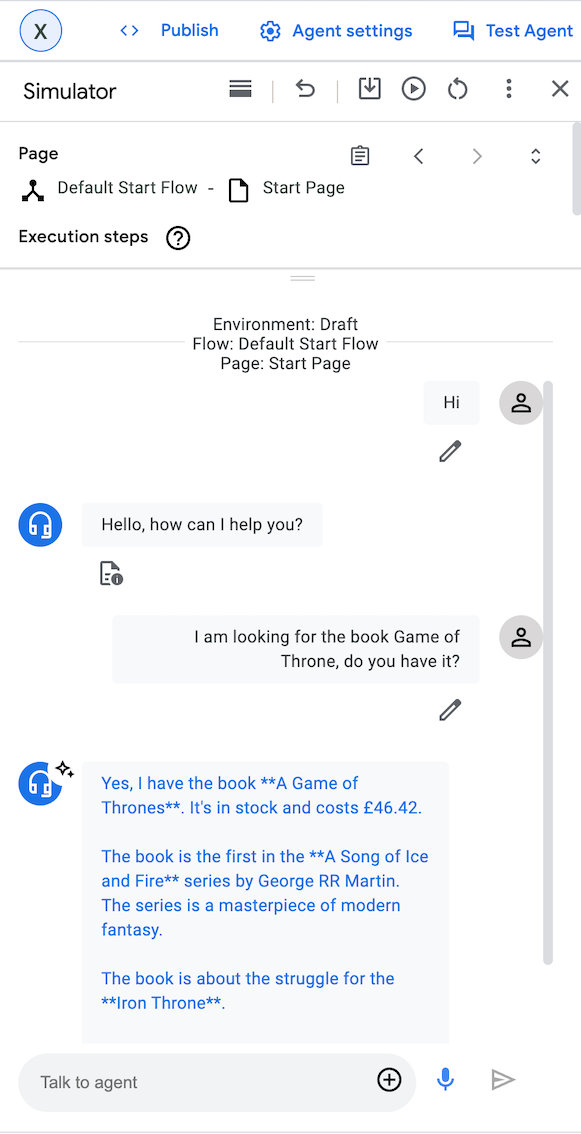
It works!
Step 5: Publish / Integrate your Chatbot (low code)
If you are happy with the Chatbot, it is easy to integrate it with your web application
Go to the left pane, select “Manage” -> “Integrations” -> “Dialogflow Messenger”

You can choose the type of API and UI style according to your needs
For demo purpose, I selected “Unauthenticated API” as API and “Pop-out” as UI style:
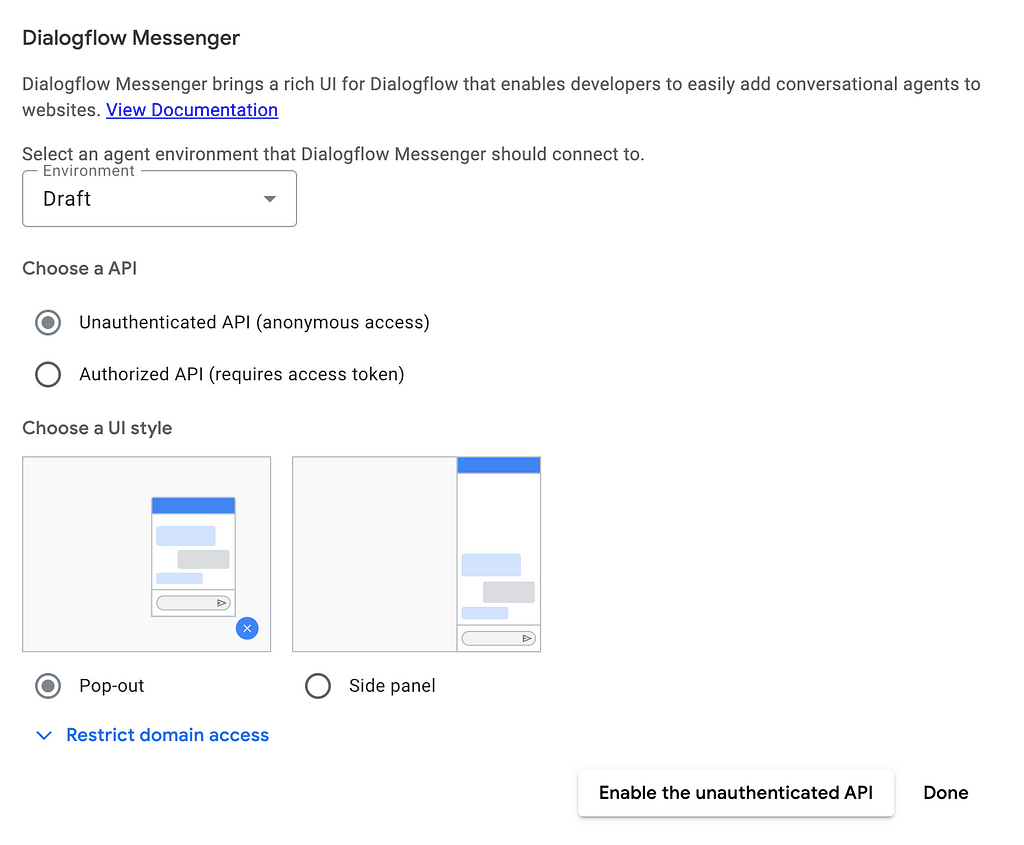
After selecting “Done”, a code snippet in HTML will be generated in the next page as below:
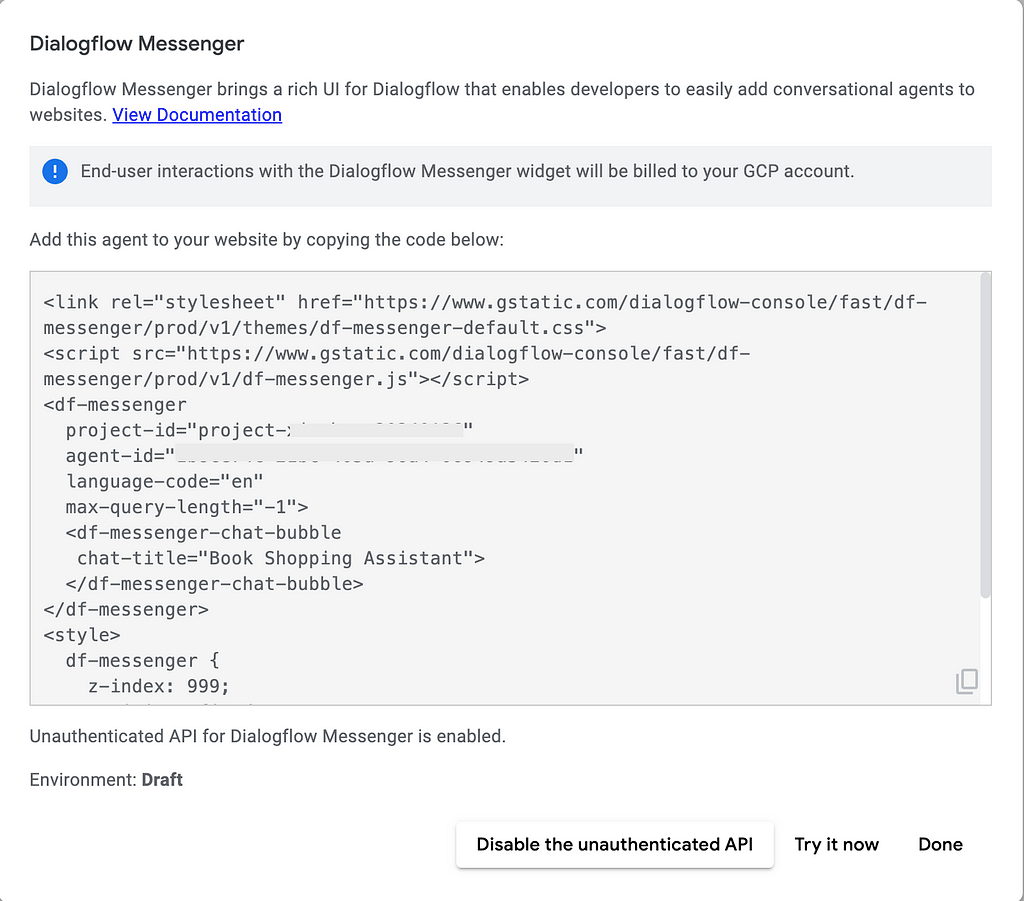
You may copy the code snippet and easily paste it into your applications for integration.
For demo purpose, I copy paste this HTML snippet into JSFiddle and run it, then I am getting my little Chatbot working as shown in the right down corner!
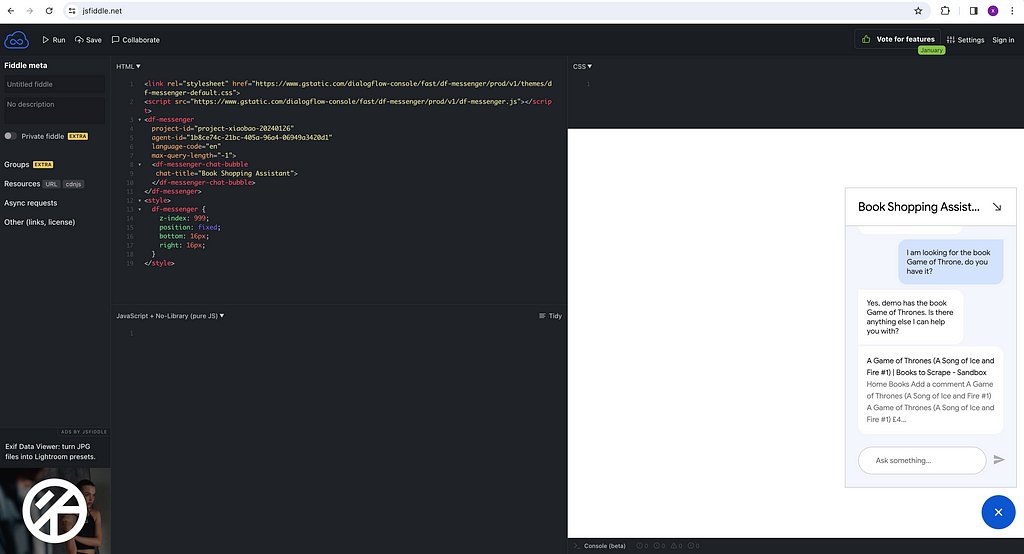
Step 6 (Optional): Publish it through a Beautiful Application (low code)
In case you don’t have an application yet and you want to have one, Google provides a good starting point through a public git repository Chat App.
This is a Chatbot Application written in Node.js and you can easily adapt it for your own use by changing the code snippets a bit within chat-app/src/routes/+page.svelte .
You will need to change the project-id, agent-id and chat-title into yours.
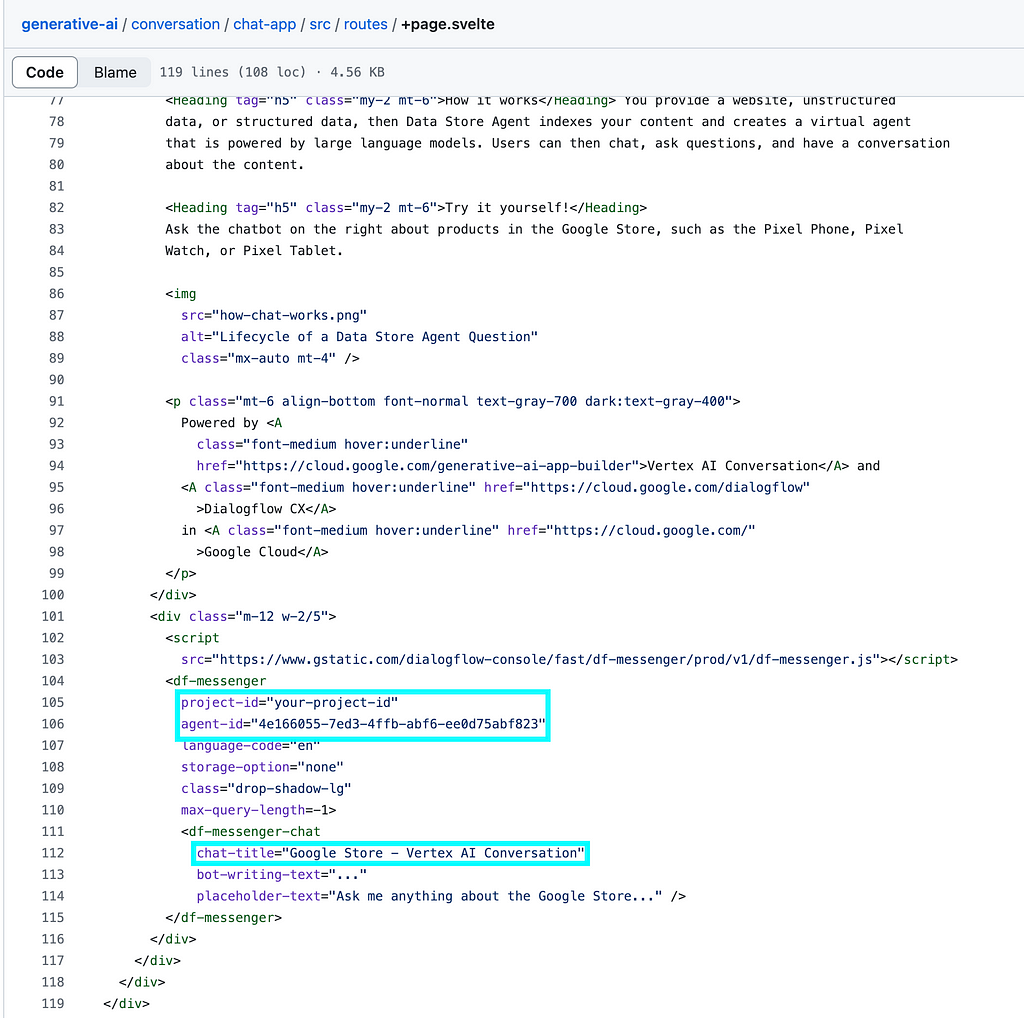
And once you run/deploy the app, you will get the web UI like this:
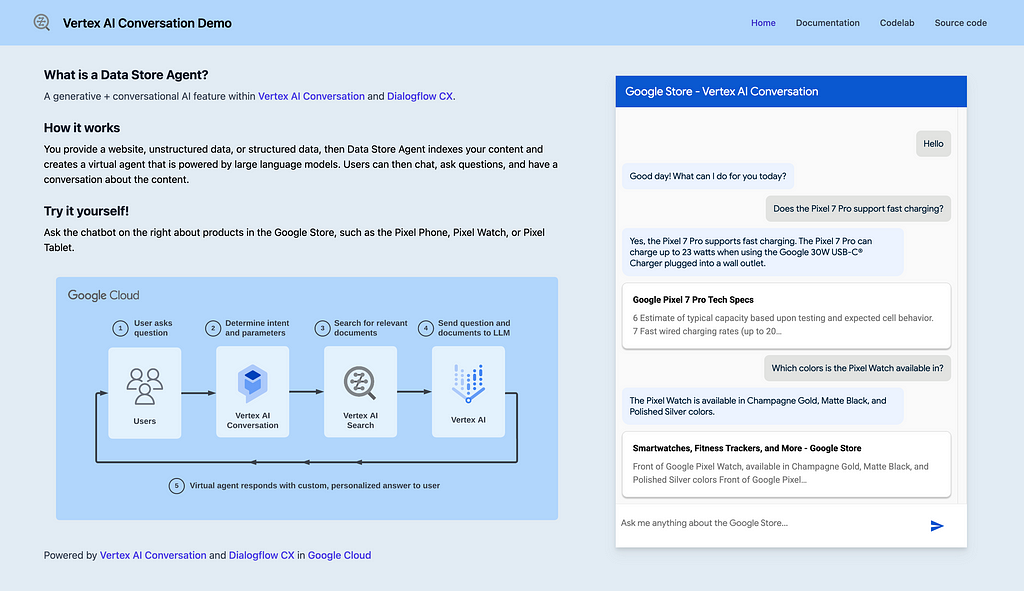
Of course you can change the appearance of the UI as you like.
Now you can have your own application!
What makes this “magic”?
Recalling the solution design that we mentioned at the beginning. This looks a bit magic as you can get your own LLM powered Chatbot by simply supplying your private knowledge to a Google Cloud Storage bucket.

This is achieved as Google has done quite a bit of integrations behind the scene, by integrating Vertex AI platform with the chatbot agent service Dialogflow CX, and coming up with a new abstraction called Vertex AI Conversation (formerly Gen App Builder). This new abstraction also supports Search and Recommend, and the full name of this service is “Vertex AI Search and Conversation”.
As we can see, this new abstraction of “Vertex AI Search and Conversation” is sitting on top of Vertex AI which has orchestrated a bunch of foundation models, and gets “augmented” by user-supplied updated real world information, so it can contextualize its responses to these information.
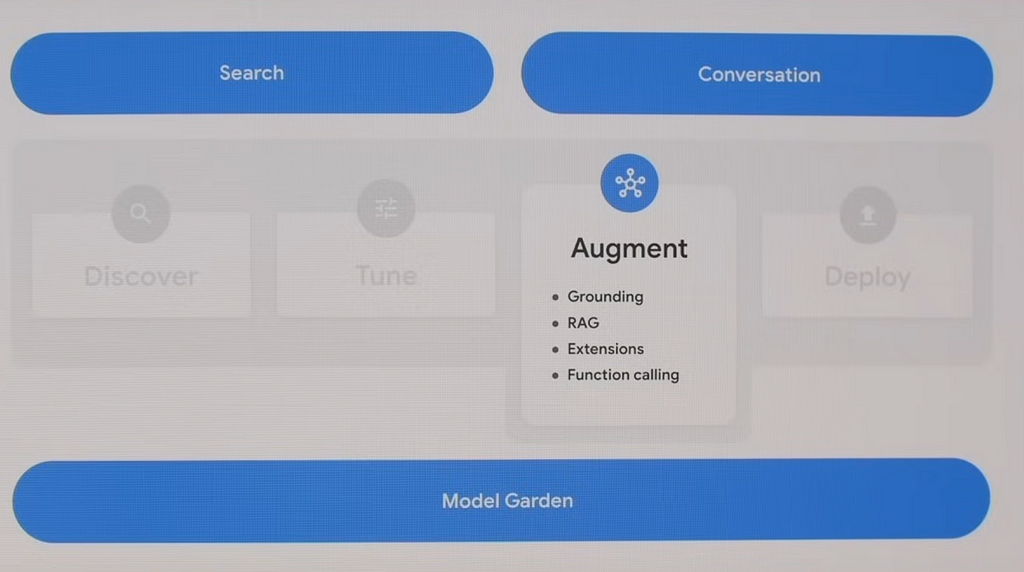
The integration is great as it can help at least two groups of people —
- traditional Chatbot builder, and
- people exploring GenAI solutions but having not identified a good use case
Imagine you are a traditional Chatbot builder using Dialogflow CX, you are creating pages, intents and routes to route customer intentions to the corresponding page. Basically you are defining “if customer say this then I respond with this” which is a bit hard-coding. Now Google plugs in Vertex AI which can utilise LLM models (e.g. text-bison, gemini) to generate agent responses and control conversation flow in a much smarter way. This can significantly reduce agent design time and improve agent quality.
On the other hand, image you are exploring the power of LLMs and Generative AI but not sure what to do with it. This Vertex AI Conversation feature can enable you to easily build and launch your own Chatbot applications quickly and make them available for real use case. This can significantly shorten the go-to-market time of LLM and GenAI solutions.
Some Observations
Despite the “magic” as it appears, we observed several things worth sharing with developers who are considering use this “Vertex AI Search and Conversation” feature.
Our gut feeling is this is a new product Google brought in by “integrating” several existing tools and is still working towards making it better. It lacks clarity how the integration happens behind the scene, and how developers can best understand and configure it.
I got our chatbot very quickly but once I started looking at how to fine tune it, it took me quite a bit of time to figure out how Dialogflow CX works, what is “generator” and how it works. At this moment I’m still confused why this Chatbot works so great without me even configuring any “generator” as described in Google doc, and whether/how we can make it better by using “generator”.
Some other observations during the development:
- Indexing a website or a set of documents can take minutes or days, depending on the amount of data. There is no clear estimate on how long this process will take, and what developers can do is just wait and check periodically.
- We know how to link a datastore to a Chatbot app, but looks like we cannot “unlink” it.
- Despite the level of grounding, the quality of the data supplied by users can significantly impact the performance of the Chatbot. “Rubbish in, rubbish out” still applies to a great extent.
- “Augment” by supplying private data and knowledge helps resolve one issue of LLM — lack of updated real world information. But the issue of hallucination stays as sometimes the Chatbot can give “fake” information (of course depending on the data quality of the private knowledge you supplied).
- The Chatbot provides links to the relevant web page / document page (e.g. PDF) during chat with users. This is great, but the link supplied by the Chatbot comes as Google Cloud Storage Authenticated URL and can only be accessed by users with granted permission. Developers need to figure out how to make them into the signed-URLs which are safe to share with public anonymous users, instead of using the Google Cloud Storage Authenticated URLs.
- The data store sitting behind the Chatbot works best for unstructured data. For structured data, it supports linking to CSV structured data but it has to be in the format of “question” and “answer” as mentioned in Google doc:

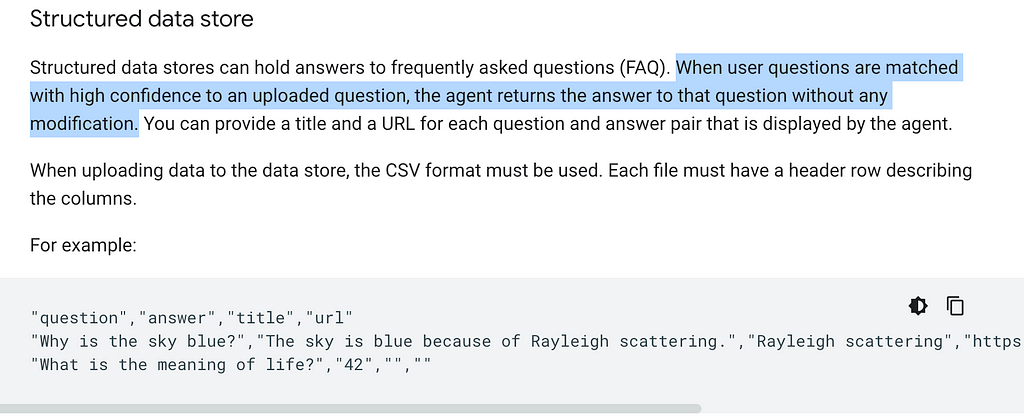
Wrap-up
In the above use case, I assume I am an online book store owner and created a Chatbot based on my e-commerce website contents in HTML.
Similarly, you can supply “private knowledge” in the format of blogs, files (e.g. PDF, HTML, TXT) and all kinds of websites to the Google Cloud Storage, and create your own Chatbot.
This enables individuals / businesses to fully utilize the power of the Google LLMs (text-bison, gemini, etc.) and augment it with private knowledge, and create own Chatbots in a very quick manner.
This marks the end of this article. Hope you find it helpful!
(PS: I am working on a video to make this step-by-step guide easier to follow. Will share if I get it done in near future.)
Enjoyed This Story?
Selina Li (Selina Li, LinkedIn) is a Principal Data Engineer working at Officeworks in Melbourne Australia. Selina is passionate about AI/ML, data engineering and investment.
Jason Li (Tianyi Li, LinkedIn) is a Full-stack Developer working at Mindset Health in Melbourne Australia. Jason is passionate about AI, front-end development and space related technologies.
Selina and Jason would love to explore technologies to help people achieve their goals.
Unless otherwise noted, all images are by the authors.
How to build your own Google AI Chatbot within 5 minutes? was originally published in Towards Data Science on Medium, where people are continuing the conversation by highlighting and responding to this story.
Originally appeared here:
How to build your own Google AI Chatbot within 5 minutes?
Go Here to Read this Fast! How to build your own Google AI Chatbot within 5 minutes?