A Quantitatively Accurate and Clinically Useful Generative Medical Imaging Model
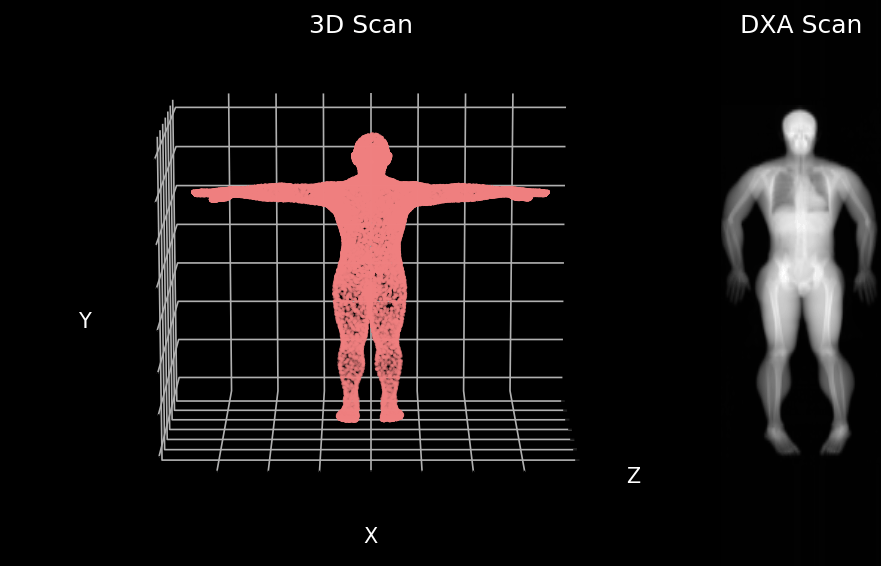
Key Points
- To our knowledge, this is the first quantitatively accurate model in which generated medical imaging can be analyzed with commercial clinical software.
- Being able to predict interior distributions of fat, muscle, and bone from exterior shape, indicates the strong relationship between body composition and body shape
- This model represents a significant step towards accessible health monitoring, producing images that would normally require specialized, expensive equipment, trained technicians, and involve exposure to potentially harmful ionizing radiation.
- Read the paper HERE
Generative artificial intelligence (AI) has become astonishingly popular especially after the release of both diffusion models like DALL-E and large language models (LLM) like ChatGPT. In general, AI models are classified as “generative” when the model produces something as an output. For DALL-E the product output is a high-quality image while for ChatGPT the product or output is highly structured meaningful text. These generative models are different than classification models that output a prediction for one side of a decision boundary such as cancer or no cancer and these are also different from regression models that output numerical predictions such as blood glucose level. Medical imaging and healthcare have benefited from AI in general and several compelling use cases and generative models are constantly being developed. A major barrier to clinical use of generative AI models is a lack of validation of model outputs beyond just image quality assessments. In our work, we evaluate our generative model on both a qualitative and quantitative assessment as a step towards more clinically relevant AI models.
Quality vs Quantity
In medical imaging, image quality is crucial; it’s all about how well the image represents the internal structures of the body. The majority of the use cases for medical imaging is predicated on having images of high quality. For instance, X-ray scans use ionizing radiation to produce images of many internal structures of the body and quality is important for identifying bone from soft tissue or organs as well as identifying anomalies like tumors. High quality X-ray images result in easier to identify structures which can translate to more accurate diagnosis. Computer vision research has led to the development of metrics meant to objectively measure image quality. These metrics, which we use in our work, include peak signal to noise ratio (PSNR) and structural similarity index (SSIM), for example. Ultimately, a high-quality image can be defined as having sharp, well defined borders, with good contrast between different anatomical structures.
Images are highly structured data types and made up of a matrix of pixels of varying intensities. Unlike natural images as seen in the ImageNet dataset consisting of cars, planes, boats, and etc. which have three red, green, and blue color channels, medical images are mostly gray scale or a single channel. Simply put, sharp edges are achieved by having pixels near the borders of structures be uniform and good contrast is achieved when neighboring pixels depicting different structures have a noticeable difference in value from one another. It is important to note that the absolute value of the pixels are not the most important thing for high quality images and it is in fact more dependent on the relative pixel intensities to each other. This, however, is not the case for achieving images with high quantitative accuracy.

A subset of medical imaging modalities is quantitative meaning the pixel values represent a known quantity of some material or tissue. Dual energy X-ray Absorptiometry (DXA) is a well known and common quantitative imaging modality used for measuring body composition. DXA images are acquired using high and low energy X-rays. Then a set of equations sometimes refered to as DXA math is used to compute the contrast and ratios between the high and low energy X-ray images to yield quantities of fat, muscle, and bone. Hence the word quantitative. The absolute value of each pixel is important because it ultimately corresponds to a known quantity of some material. Any small changes in the pixel values, while it may still look of the same or similar quality, will result in noticeably different tissue quantities.
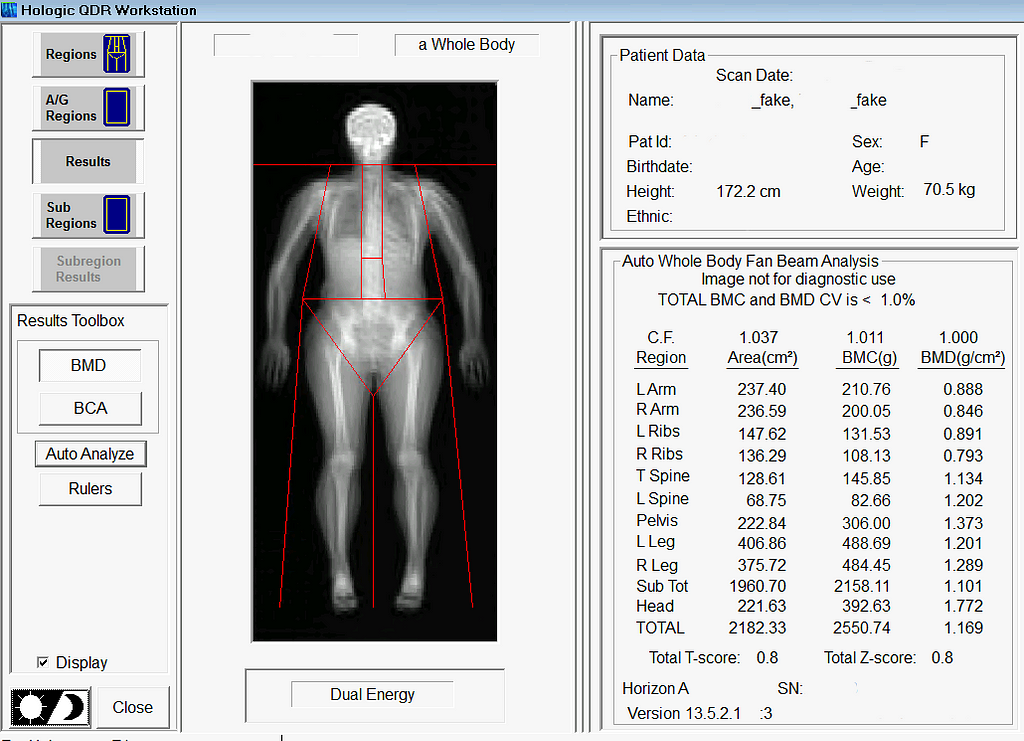
Generative AI in Medical Imaging
As previously mentioned, generative AI models for medical imaging are at the forefront of development. Known examples of generative medical models include models for artifact removal from CT images or the production of higher quality CT images from low dose modalities where image quality is known to be lesser in quality. However, prior to our study, generative models creating quantitatively accurate medical images were largely unexplored. Quantitative accuracy is arguably more difficult for generative models to achieve than producing an image of high quality. Anatomical structures not only have to be in the right place, but the pixels representing their location needs to be near perfect as well. When considering the difficulty of achieving quantitative accuracy one must also consider the bit depth of raw medical images. The raw formats of some medical imaging modalities, DXA included, encode information in 12 or 14 bit which is magnitudes more than standard 8-bit images. High bit depths equate to a bigger search space which could equate to it being more difficult to get the exact pixel value. We are able to achieve quantitative accuracy through self-supervised learning methods with a custom physics or DXA informed loss function described in this work here. Stay tuned for a deep dive into that work to come in the near future.
What We Did
We developed a model that can predict your insides from your outsides. In other words, our model innovatively predicts internal body composition from external body scans, specifically transforming three-dimensional (3D) body surface scans into fully analyzable DXA scans. Utilizing increasingly common 3D body scanning technologies, which employ optical cameras or lasers, our model bypasses the need for ionizing radiation. 3D scanning enables accurate capture of one’s exterior body shape and the technology has several health relevant use cases. Our model outputs a fully analyzable DXA scan which means that existing commercial software can be used to derive body composition or measures of adipose tissue (fat), lean tissue (muscle), and bone. To ensure accurate body composition measurements, our model was designed to achieve both qualitative and quantitative precision, a capability we have successfully demonstrated.
Inspiration and Motivation
The genesis of this project was motivated by the hypothesis that your body shape or exterior phenotype is determined by the underlying distribution of fat, muscle, and bone. We had previously conducted several studies demonstrating the associations of body shape to measured quantities of muscle, fat, and bone as well as to health outcomes such as metabolic syndrome. Using principal components analysis (PCA), through shape and appearance modeling, and linear regression, a student in our lab showed the ability to predict body composition images from 3D body scans. While this was impressive and further strengthened the notion of the relationship between shape and composition, these predicted images excluded the forelimbs (elbow to hand and knee to feet) and the images were not in a format (raw DXA format) which enabled analysis with clinical software. Our work fully extends and overcomes previous limitations. The Pseudo-DXA model, as we call it, is able to generate the full whole body DXA image from 3D body scan inputs which can be analyzed from using clinical and commercial software.

Our Training Data
The cornerstone of the Pseudo-DXA model’s development was a unique dataset comprising paired 3D body and DXA scans, obtained simultaneously. Such paired datasets are uncommon, due to the logistical and financial challenges in scanning large patient groups with both modalities. We worked with a modest but significant sample size: several hundred paired scans. To overcome the data scarcity issue, we utilized an additional, extensive DXA dataset with over 20,000 scans for model pretraining.
Building the Model
The Pseudo-DXA model was built in two steps. The first self-supervised learning (SSL) or pretraining step involved training a variational auto encoder (VAE) to encode and decode or regenerate raw DXA scan. A large DXA data set, which is independent of the data set used in the final model and evaluation of our model, was used to SSL pretrain our model and it was divided to contain an separate hold out test set. Once the VAE model was able to accurately regenerate the original raw DXA image as validated with the holdout test set, we moved to the second phase of training.
In brief, VAE models consist of two main subnetwork components which include the encoder and the decoder, also known as a generator. The encoder is tasked with taking the high dimensional raw DXA image data and learning a meaningful compressed representation which is encoded into what is known as a latent space. The decoder or generator takes the latent space representation and learns to regenerate the original image from the compressed representation. We used the trained generator from our SSL DXA training as the base of our final Pseudo-DXA model.
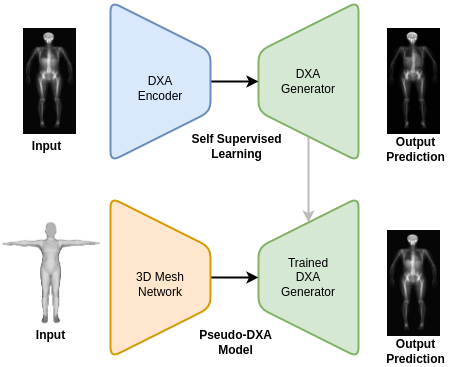
The structure of the 3D body scan data consisted of a series of vertices or points and faces which indicate which points are connected to one another. We used a model architecture resembling the Pointnet++ model which has demonstrated the ability to handle point cloud data well. The Pointnet++ model was then attached to the generator we had previously trained. We then fed the mode the 3D data and it was tasked with learning generate the corresponding DXA scan.
Pseudo-DXA Results
In alignment with machine learning best practices, we divided our data such that we had an unseen holdout test for which we reported all our results on.
Image quality
We first evaluated our Pseudo-DXA images using image quality metrics which include normalized mean absolute error (NMAE), peak signal to noise ratio (PSNR), and structural similarity index (SSIM). Our model generated images had mean NMAE, PSNR, and SSIM of 0.15, 38.15, and 0.97, respectively, which is considered to be good with respect to quality. Shown below is an example of a 3D scan, the actual DXA low energy scan, Pseudo-DXA low energy scan and the percent error map of the two DXA scans. As mentioned DXA images have two image channels for high and low energies yet, these examples are just showing the low energy image. Long story short, the Pseudo-DXA model can generate high quality images on par with other medical imaging models with respect to the image quality metrics used.
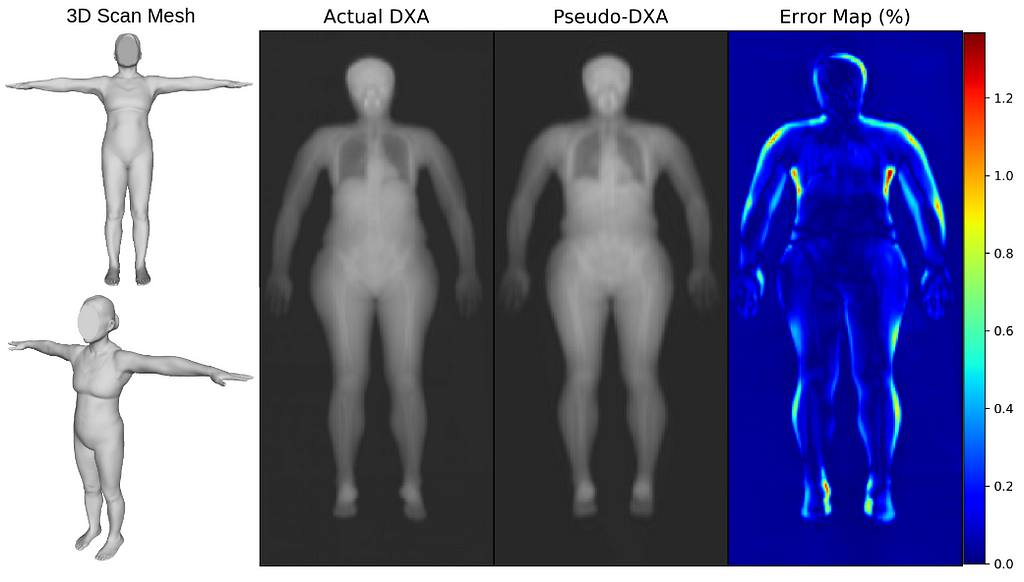
Quantitative Accuracy
When we analyzed our Pseudo-DXA images for composition and compare the quantities to the actual quantities we achieved coefficients of determination (R²) of 0.72, 0.90, 0.74, and 0.99 for fat, lean, bone, and total mass, respectively. An R²of 1 is desired and our values were reasonably close considering the difficulty of the task. A comment we encountered when presenting our preliminary findings at conferences was “wouldn’t it be easier to simply train a model to predict each measured composition value from the 3D scan so the model would for example, output a quantity of fat and bone and etc., rather than a whole image”. The short answer to the question is yes, however, that model would not be as powerful and useful as the Pseudo-DXA model that we are presenting here. Predicting a whole image demonstrates the strong relationship between shape and composition. Additionally, having a whole image allows for secondary analysis without having to retrain a model. We demonstrate the power of this by performing ad-hoc body composition analysis on two user defined leg subregions. If we had trained a model to just output scalar composition values and not an image, we would only be able to analysis these ad-hoc user defined regions by retraining a whole new model for these measures.
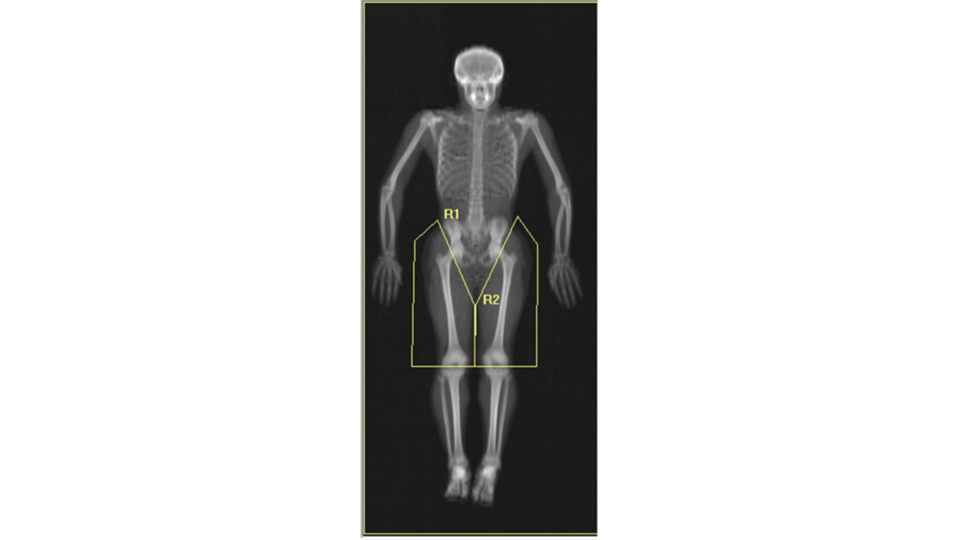
Long story short, the Pseudo-DXA model produced high quality images that were quantitatively accurate, from which software could measure real amounts of fat, muscle, and bone.
So What Does This All Mean?
The Pseudo-DXA model marks a pivotal step towards a new standard of striving for quantitative accuracy when necessary. The bar for good generative medical imaging models was high image quality yet, as we discussed, good quality may simply not be enough given the task. If the clinical task or outcome requires something to be measured from the image beyond morphology or anthropometry, then quantitative accuracy should be assessed.
Our Pseudo-DXA model is also a step in the direction of making health assessment more accessible. 3D scanning is now in phones and does not expose individuals to harmful ionizing radiation. In theory, one could get a 3D scan of themselves, run in through our models, and receive a DXA image from which they can obtain quantities of body composition. We acknowledge that our model generates statistically likely images and it is not able to predict pathologies such as tumors, fractures, or implants, which are statistically unlikely in the context of a healthy population from which this model was built. Our model also demonstrated great test-retest precision which means it has the ability to monitor change over time. So, individuals can scan themselves every day without the risk of radiation and the model is robust enough to show changes in composition, if any.
We invite you to engage with this groundbreaking technology and/or provided an example of a quantitatively accurate generative medical imaging model. Share your thoughts, ask questions, or discuss potential applications in the comments. Your insights are valuable to us as we continue to innovate in the field of medical imaging and AI. Join the conversation and be part of this exciting journey!
More Resources
Read The Paper
Model and Data Request
- LambertLeong/Pseudo-DXA: v1.0.1
- GitHub – LambertLeong/Pseudo-DXA: Generative medical imaging model that produces real DXA scan from 3D body surface scan inputs. Predicting interior fat, lean, and bone distribution fom exterior body shape.
- Data & Software – Shepherd Research Lab
AI Predicts Your Insides From Your Outsides With Pseudo-DXA was originally published in Towards Data Science on Medium, where people are continuing the conversation by highlighting and responding to this story.
Originally appeared here:
AI Predicts Your Insides From Your Outsides With Pseudo-DXA
Go Here to Read this Fast! AI Predicts Your Insides From Your Outsides With Pseudo-DXA