A pragmatic guide to understand AI risks and build trust with users through responsible AI development
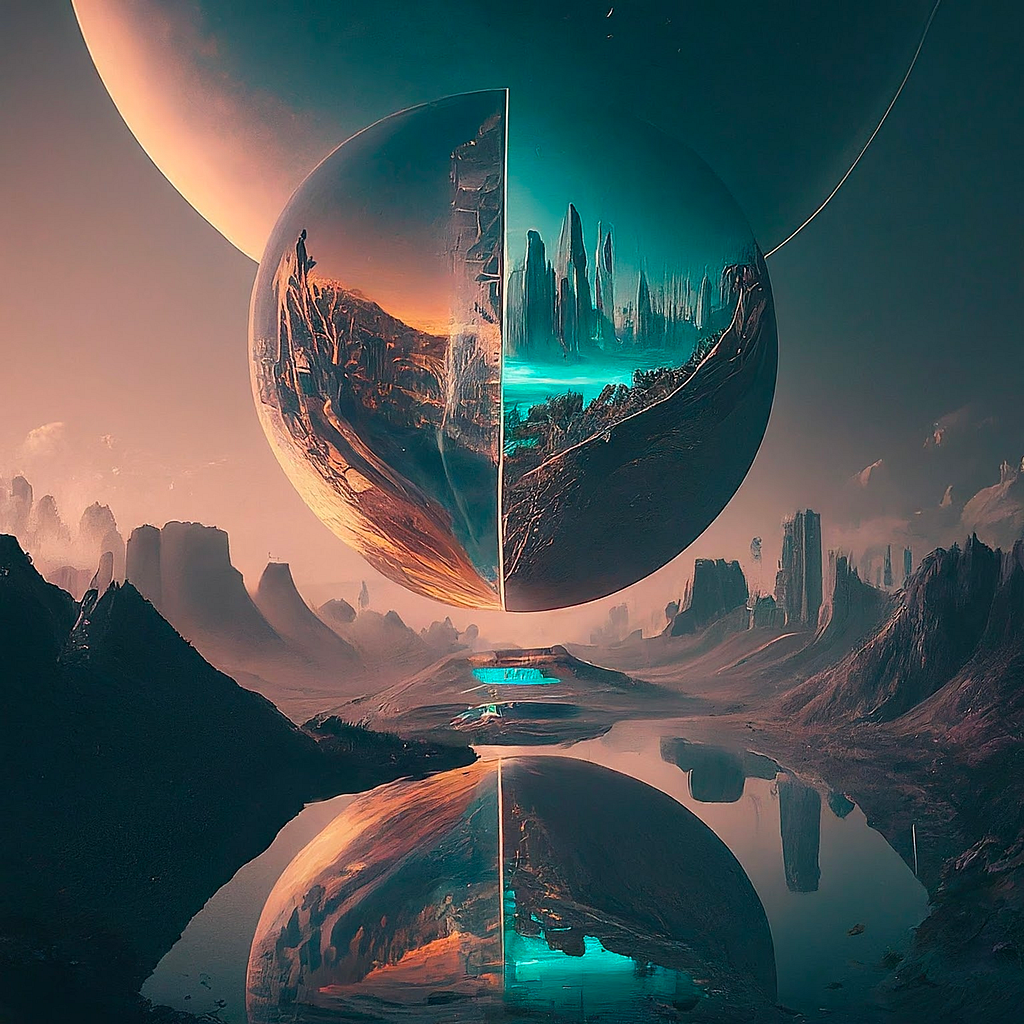
In the fast-paced technology landscape, product teams feel the relentless pressure to rush innovative AI offerings to market. However, prioritizing speed over ethical and safety considerations can severely backfire. When AI systems breach social norms and values, organizations bear the risk of facing long-lasting reputational damage and losing trust with users.
Companies are caught in a real-world “prisoner’s dilemma” of the AI era — while collective adherence to ethical development is ideal, it’s often more tempting for companies to secure a first-mover advantage by compromising on these crucial standards. This tension is exemplified by the infamous Facebook-Cambridge Analytica scandal, where taking ethical shortcuts led to grave, long-lasting reputational damage. As AI becomes more entrenched in our daily lives, the stakes of this ethical gamble only heightens, with each player in the industry weighing immediate gains against the longer-term value of trust and responsibility.
With the rise in AI adoption across industries since 2018, high-profile incidents of ethical violations have sharply increased. Mapping out these risks and mitigation strategies is now pivotal. This article probes the key source of AI risks, examines a few high-profile fallouts, and lays out a 6 steps framework for building products that counter these threats.
Identifying the Risks of AI
1. Privacy Intrusion & Consent Issue
Risk: Arguably the most prevalent AI pitfall is the infringement of privacy and copyright laws. This entails two distinct types of failures: failure to secure consent for the use of data, and using the data for purposes beyond the scope for which the consent was given.
Example: Artists and writers have filed class action lawsuits against OpenAI and MidJourney for training models with their work without consent. Similarly, Getty Images is suing Stability AI for using its data for model training. Privacy breaches can also occur even when consent is given but the data is used for unintended purposes. For example, Google DeepMind used the data of 1.6 million patients at the Royal London NHS Foundation Trust to build a new healthcare application. Although there was an implied consent that the data could be used to improve patients’ health, the Trust and DeepMind did not clearly communicate to patients that their information is being used to build an app.
- Artists take new shot at Stability, Midjourney in updated copyright lawsuit
- Why Google consuming DeepMind Health is scaring privacy experts
2. Algorithmic Bias
Risk: This risk involves AI systems making biased predictions, which systematically disadvantages or excludes certain group of people based on characteristics like race, gender, or socio-economic background. Such biases can have significant societal impact, especially when the AI is used to make critical decisions impacting the lives of the individuals.
Example: There was backlash against Apple in 2019, when allegations surfaced that men received higher credit limits for Apple Credit Cards than women, despite women having better credit scores in some cases. Other notable instances include AI-driven recruitment software and criminal justice applications, such as the COMPAS tool, which have been criticized for displaying racial and gender bias.
- Apple Card is accused of gender bias. Here’s how that can happen | CNN Business
- Opinion | When an Algorithm Helps Send You to Prison (Published 2017)
3. Accuracy Risk & Explainability Gap
Risk: Significant risks arise when AI systems that are used for making high-stakes decisions provide inaccurate results or fail to offer a clear rationale for their output. The ‘black box’ nature of the AI makes it difficult for users to understand and verify its results, which obscures accountability and leads to a loss of trust.
Example: IBM Watson for Oncology was designed to provide clinicians with personalized recommendations for cancer treatment. However, reports surfaced that Watson gave unsafe and incorrect treatment suggestions, which led to a loss of trust in technology and damaged IBM’s reputation.
4. Security Risk
Risk: A significant societal risks emerge from the use of AI to create deepfakes, which involves generating highly convincing images or video that makes it appear as though individuals are saying or doing things they never actually did. Deepfakes have been used to create deceptive and realistic media that can be used for committing fraud, spreading misinformation, or damaging reputations. Moreover, AI can be weaponized for cyberattacks and social engineering, like tailoring phishing campaigns, which can introduce extensive security vulnerabilities.
Example: In the political domain, use of deepfakes to fabricate speeches or actions could sway public opinion during critical events like elections. During the Russia-Ukraine conflict, a deepfake showing Ukrainian president appearing to tell his soldiers to lay down arms and surrender was circulated, an act that could have demoralized troops and provided time-sensitive tactical advantage to Russia. In the cybersecurity realm, AI-powered voice imitation was used to impersonate a CEO’s voice convincingly, leading to a fraudulent transfer of funds.
- Deepfake video of Zelenskyy could be ‘tip of the iceberg’ in info war, experts warn
- Unusual CEO Fraud via Deepfake Audio Steals US$243,000 From UK Company
6 Step Framework for Mitigating AI Risks
Managing AI risks requires a thoughtful approach throughout the entire product life-cycle. Below is a six step framework, organized by different stages of AI development, that organizations can adopt to ensure the responsible use of AI technology in their products.
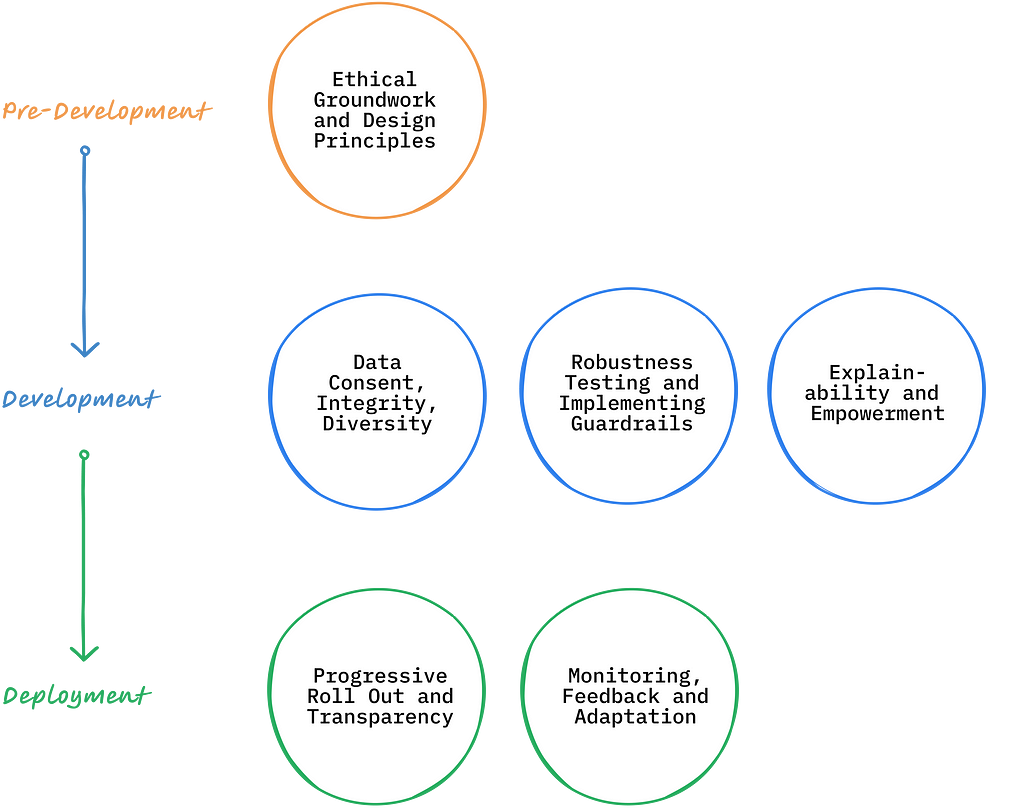
1. Pre-Development: Ethical Groundwork and Design Principles
Before a single line of code is written, product teams should lay out the groundwork. Prioritize early engagement with a broad set of stakeholders, including users, technical experts, ethicists, legal professionals, and members of communities who may be impacted by the AI application. The goal is to identify both the overt and subtle risks associated with the product’s use case. Use these insights to chalk out the set of ethical guidelines and product capabilities that needs to be embedded into the product prior to its launch to preemptively address the identified risks.
2. Development: Data Consent, Integrity, Diversity
Data is the bedrock of AI and also the most significant source of AI risks. It is critical to ensure that all data procured for model training are ethically sourced and comes with consent for its intended use. For example, Adobe trained its image generation model (Firefly) with proprietary data which allows it to provide legal protection to users against copyright lawsuits.
Further, Personally Identifiable Information (PII) should be removed from sensitive datasets used for training models to prevent potential harm. Access to such datasets should be appropriately gated and tracked to protect privacy. It’s equally important to ensure that the datasets represent the diversity of user base and the breadth of usage scenarios to mitigate bias and fairness risks. Companies like Runway have trained their text-to-image models with synthetic datasets containing AI-generated images of people from different ethnicities, genders, professions, and ages to ensure that their AI models exhibit diversity in the content they create.
- How Adobe’s Copyright Protection Will Make it an AI Leader
- Mitigating stereotypical biases in text to image generative systems | Runway Research
3. Development: Robustness Testing and Implementing Guardrails
The testing phase is pivotal in determining AI’s readiness for a public release. This involves comparing AI’s output against the curated set of verified results. An effective testing uses:
- Performance Metrics aligned with user objectives and business values,
- Evaluation Data representing users from different demographics and covering a range of usage scenarios, including edge-cases
In addition to performance testing, it is also critical to implement guardrails that prevents AI from producing harmful results. For instance, ImageFX, Google’s Image generation service, proactively blocks users from generating content that could be deemed inappropriate or used to spread misinformation. Similarly, Anthropic has proactively set guardrails and measures to avoid misuse of its AI services in 2024 elections.
- New and better ways to create images with Imagen 2
- Anthropic on LinkedIn: Preparing for global elections in 2024
4. Development: Explainability & Empowerment
In critical industry use cases where building trust is pivotal, it’s important for the AI to enable humans in an assistive role. This can be achieved by:
- Providing citations for the sources of the AI’s insights.
- Highlighting the uncertainty or confidence-level of the AI’s prediction.
- Offering users the option to opt-out of using the AI.
- Creating application workflows that ensure human oversight and prevent some tasks from being fully automated.
5. Deployment: Progressive Roll Out & Transparency
As you transition the AI systems from development to real-world deployment, adopting a phased roll-out strategy is crucial for assessing risks and gathering feedback in a controlled setting. It’s also important to clearly communicate the AI’s intended use case, capabilities, and limitations to users and stakeholders. Transparency at this stage helps manage expectations and mitigates reputational risks associated with unexpected failures of the AI system.
OpenAI, for example, demonstrated this approach with Sora, its latest text-to-video service, by initially making the service available to only a select group of red teamers and creative professionals. It has been upfront about Sora’s capabilities as well as its current limitations, such as challenges in generating video involving complex physical interactions. This level of disclosure ensures users understand where the technology excels and where it might fail, thereby managing expectations, earning users’ trust, and facilitating responsible adoption of the AI technology.
Sora: Creating video from text
6. Deployment: Monitoring, Feedback, and Adaptation
After an AI system goes live, the work isn’t over. Now comes the task of keeping a close watch on how the AI behaves in the wild and tuning it based on what you find. Create an ongoing mechanism to track performance drifts and continually test and train the model on fresh data to avoid degradation in the AI performance. Make it easy for users to flag issues and use these insights to adapt AI and constantly update guardrails to meet high ethical standards. This will ensure that the AI systems remain reliable, trustworthy, and in step with the dynamic world they operate in.
Conclusion:
As AI becomes further entrenched in our lives, managing ethical risks proactively is no longer optional — it is imperative. By embedding ethical considerations in every step of bringing AI products to life, companies not only mitigate risks but also build foundational trust with their users. The era of “move fast and break things” cannot be applied when dealing with technologies that are exponentially more powerful than anything we have seen before. There are no shortcuts when it comes to managing risks that can have far-reaching societal impacts.
AI product builders have a duty to society to move more intentionally and purposefully, making trust their true North Star. The future success and continued progress of AI hinges on getting the ethics right today.
Thanks for reading! If these insights resonate with you or spark new thoughts, let’s continue the conversation. Share your perspectives in the comments below or connect with me on LinkedIn.
6 Step Framework to Manage Ethical Risks of Generative AI in Your Product was originally published in Towards Data Science on Medium, where people are continuing the conversation by highlighting and responding to this story.
Originally appeared here:
6 Step Framework to Manage Ethical Risks of Generative AI in Your Product
Go Here to Read this Fast! 6 Step Framework to Manage Ethical Risks of Generative AI in Your Product