How to correctly measure sales performance and identify opportunities to enhance sales strategy
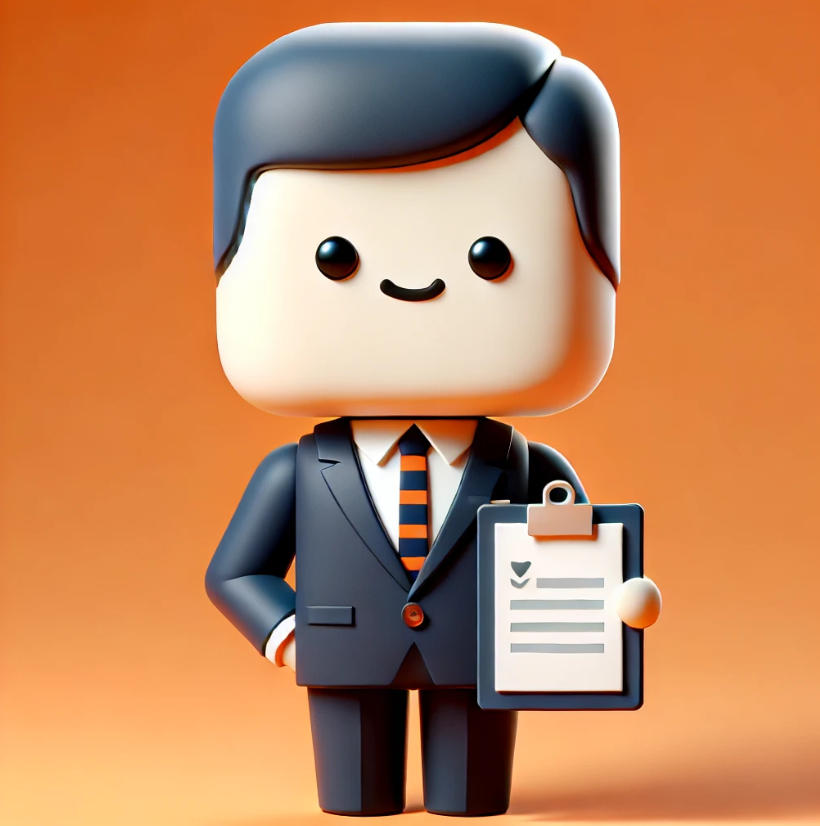
Throughout my career, I have collaborated with numerous companies that believed they had a clear understanding of which sales agents were their top performers and which ones were underperforming.
However, I can state that 9 out of 10 were wrong.
They were misled for years due to one simple reason: they measured sales performance incorrectly.
I can also guarantee that 100% of them, after learning the correct way to measure sales performance, they have completely changed their approach and have never measured it the same way again.
Moreover, some of them have even modified their bonus compensation based on this new method of calculating sales performance.
But, what were they getting wrong?
Let’s see it with a clear example:

The table shows the annual summary of leads handled, sales, and conversion for each sales agent of a B2C company that sell a single type of product.
Faced with these results, I have typically encountered two types of interpretation:
- Those who consider the best agent to be “CL103.” This interpretation is based on the absolute number of sales or revenue (2,708 total sales). And while estimating sales performance by revenue is what intuition first tells us, this way of measuring is biased. The problem is that this agent has managed a larger number of leads. Therefore, having had access to more potential customers and having worked more, he has undoubtedly had more opportunities than the others, and thus, it is normal that he has converted more.
- Those who consider the best agent to be “AG101.” This interpretation is based on conversion rate (7.90%). In this case, the previous bias is normalized. Conversion rate compares the agents’ ability to generate sales, regardless of the number of leads each agent has received. In other words, it evaluates the transformation of leads into sales, regardless of the initial absolute number of leads, providing a more equitable view of each agent’s performance. However, as we see, agent “AG101” has provided less absolute sales. Sometimes, agents with a less volume of leads can focus on converting them and provide better service, but in that case, the owner AG101 has the aproximately the same volume as MJ105 or IG105, and significantly overperforms them.
What if I told you that neither of these criteria is really optimal? Is it possible that they are overlooking something?
The answer is YES. They are overlooking a very relevant factor: the quality of the leads received.
Lead Quality
Is it fair to evaluate in the same way a sales agent who receives leads with high interest in the product, compared to another who receives leads with low interest?
Obviously, it would not.
Agents who handle highly qualified willing-to-buy leads will naturally have better results in terms of sales and conversion, compared to those who receive leads that require more effort and time to convert.
By not considering the quality of the leads in our analysis, we are evaluating sales agents with a limited approach.
Leads with a higher probability to convert are an easier task than those with lower probability. Therefore, to obtain a fairer and more accurate evaluation of agents’ performance, it is necessary to consider the quality of the leads in the analysis as a grade of difficulty of the work performed.
This involves adding a measure of the level of interest of leads, i.e., their probability of conversion before assigning them to the agents. In this way, the performance metrics can be adjusted according to the level of effort required by each agent.
For this client, I implemented a Lead Scoring system with byRatings, based on a Machine Learning prediction model that measured the quality of the leads at the moment of their generation. This allows for an accurate comparison of lead quality at the same temporal instant.
Lead Scoring models provide the likelihood of conversion to sale for each lead. So the average Lead Score of an agent represents the expected Conversion Rate for this agent.
Let’s look at the results by adding the “Average Score” or average conversion probability of the leads received by each agent.

As we can see, the quality of the leads is a determining factor in the conversion rate. Agents like “AG101”, who we saw had the highest conversion rate, also received the highest quality leads.
Then, Average Score demonstate to be a relevant factor to consider in order to fairly compare lead assignation.
So, how can we measure sales efficiency?
Performance Ratio, the Ultimate Sales Performance Metric
As discussed above, to fairly and accurately evaluate the performance of sales agents, it is crucial to consider both the agents’ conversion rate and the quality of the leads.
A good way to calculate it is using the following formula:

This formula calculates the performance ratio, which measures the difference between the conversion rate and the average score in relation to the average score, expressed as a percentage.
A positive ratio indicates the agent is performing better than the expected conversion, while a negative ratio indicates underperformance.
Let’s look at the results again including the Performance Ratio:

The sales owner “JC102” has the highest performance ratio (110.5%), indicating that significantly outperform the average lead quality received. Despite having an average score of 1.90%, achieves a 4.00% conversion rate, showing exceptional efficiency in converting lower-quality leads into sales.
On the other hand, the sales owner “IG106” has a -57.7% indicating that even receiving leads with 2.60% of expected conversion, its perfomance is underperformed.
Final Notes
In conclusion, the performance ratio is the fairest way to measure sales performance, providing a definition of how well a sales agent converts leads relative to the average quality of those leads.
But… let’s aim for top honors.
Having a Lead Scoring model that provides the quality of leads received, can we improve the overall conversion by redistributing assignation?
For several years, I had the following hypothesis:
By providing the best leads to the best-performing agents, the overall conversion will increase.
This makes a lot of sense, because it’s likely that some opportunities are lost due to assigning good leads to agents who are less qualified or have lower sales performance.
I was repeating myself that this was the best approach, but lately I realize that I’ve also missed something.
My hypothesis was based on best agents convert best qualified leads. But, Is that true?
Let’s validate it.
One effective way to do it is by calculating the Performance Ratio on the Best Leads. Assuming that the Best Leads are the top 30% scored leads , which in that use case they had a Conversion Lift of 3.5x over the average, let’s calculate the Performance Ratio for this particular segment.
The formula is as follows:

Adding this calculation to the table, the results were as follows:

Again, JC102, who is the best agent converting overall leads (110.5% Performance Ratio), demonstrates to be also the best agent at converting the best leads (64.1% Performance Ratio of Best Leads), as we assumed in the hypothesis.
This confirm that, in this particular case, the best agent is the best converting the best leads.
However, note that the assumption is not linearly correlated for all agents.
Although “AG101” shows a strong overall performance ratio (88.1%), the negative performance ratio on the best leads (-28.8%) suggests that AG101 may not be leveraging the best leads as effectively as expected. This could indicate that AG101 could be speciallized on some sort of leads, but not necessarily the best ones.
In fact, CL103, which has a 17.7% of Performance Ratio of Best Leads, can be even a better agent to convert Best Leads than AG101 (-28.8%), although having a lower overall Performance Ratio (5.9%) than AG101 (88.1%).
Not every good agent performs well with the best leads
For those experts on sales strategy, I like you to consider that there is an even better approach to optimize the assignment, which involves evaluating each lead individually and assigning it to the agent who has the highest probability of converting it.
However, as it’s observed in the table above, in general terms the best agents handle the best leads better than the worst agents, making it a good starting point.
Further posts?
In conclusion, assigning the best leads to the best-performing agent can be a good baseline for optimizing lead allocation.
Additionally, adopting a specific agent assignment algorithm would be the most effective strategy in order to fully maximize conversion.
This can serve as an introduction to another important topic: “How to Develop the Ultimate Optimization Algorithm for Lead Assignment”.
Under such an algorithm, each lead would be assigned to the agent who can derive the greatest performance from it, i.e., who can achieve the highest conversion margin relative to the expected conversion (probability) of the lead before assignment.
In a future post, I will go into more detail about lead allocation optimization for maximizing conversion rates.
If you found this post insightful, stay tuned for more on this subject.
Unlocking Sales Performance Metrics was originally published in Towards Data Science on Medium, where people are continuing the conversation by highlighting and responding to this story.
Originally appeared here:
Unlocking Sales Performance Metrics
Go Here to Read this Fast! Unlocking Sales Performance Metrics